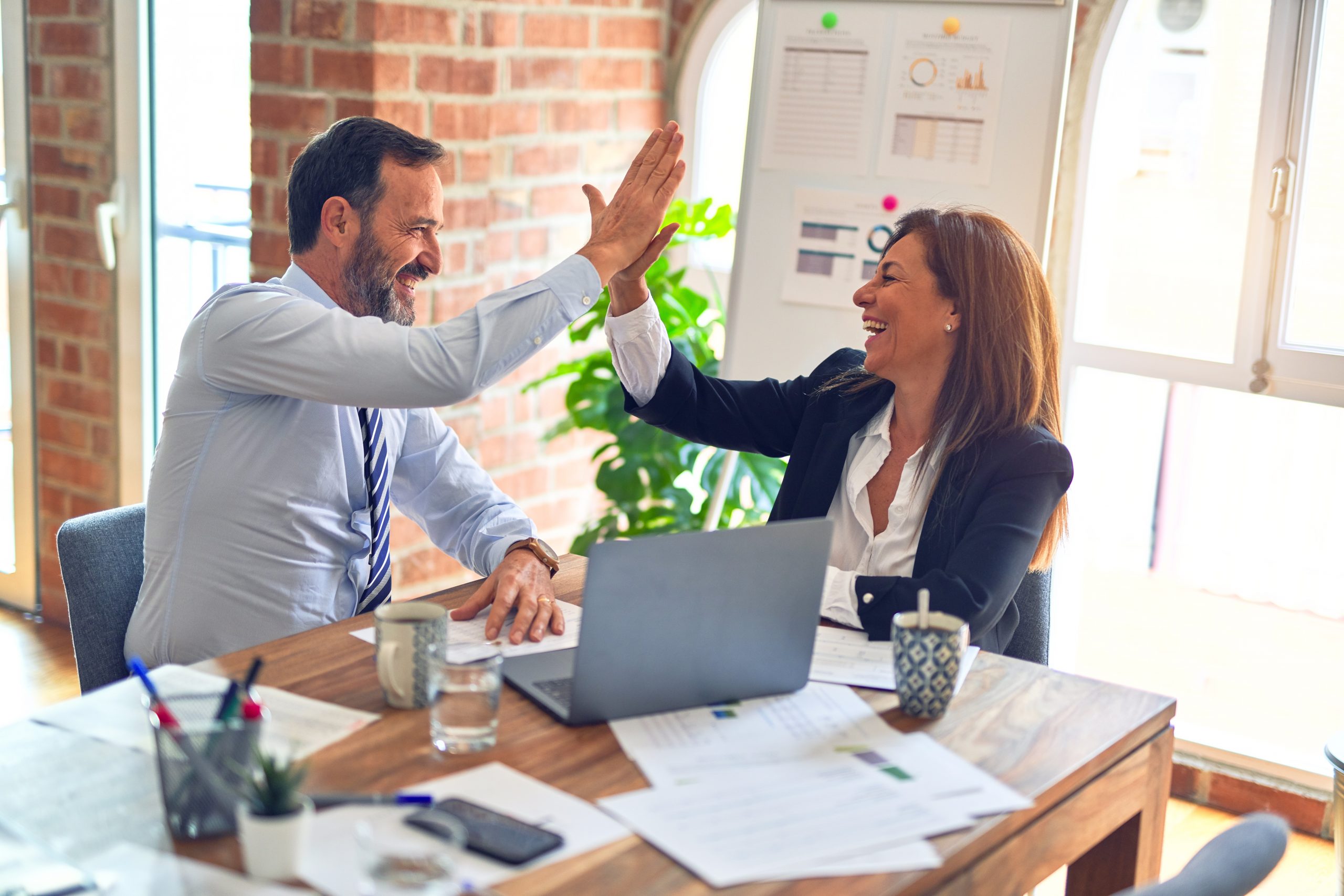
(Or “On Arithmetic Means”, if you prefer.)
I hardly need mention in this forum that as a standalone descriptive statistic, the average can be a dangerous piece of information. Averages quoted in the absence of other descriptive statistics are generally insufficient at best, and downright misleading at worst. I’ll skip the lecture on the importance of variation and related topics (Wheeler already has it nailed in this book anyway), but I do want to focus on one particular aspect of averages that I find a lot of people forget. Including me.
A lot of the time, the average never occurs.
For example, the average weight of the four residents in my household is 31 pounds. No, we aren’t long lost Lilliputians – in fact, no one in the house (me, my wife, the cat, the dog, 6 fish) weighs anything close to 31 pounds. Or to paraphrase the old joke about statisticians, on average they feel like the temperature is just fine if they are sleeping with their head in the freezer and their feet in the fire.
To a statistician or Black Belt or anyone else suitably well versed on the topic, this is hardly earth-shattering news. But to some people, the fact that a statistical average isn’t synonymous with a “usual” or “typical” value within the population is surprising. And even to those of us who know better, this fact can be a slippery one to hold on to.
Mathematically, the fact that the average might never occur in a population is not hard to understand or explain. And I don’t have trouble remembering it mathematically. The mistake I tend to make is conceptual.
Suppose, for example, you are designing a project tracking process. You might be using Excel spreadsheets and creating a home-grown solution for a dozen projects, or working with a vendor to roll out something much bigger and more complex for hundreds of projects. Either way, in my experience the tendency is to design the system for a project with “average needs” and “average complexity”. The problem is that the “average project” isn’t in your portfolio – it’s an entirely hypothetical entity. Design for it, and you’ll have a solution that perfectly fits a need that doesn’t exist.
Another example is assigning pre-work before Black Belt training. Sure, we might know what the “average person” needs to do – we’ve almost certainly designed our class for the “average participant” who has an “average background”, right? But our classes don’t fill with average people. They fill with some people who know a lot and don’t need much pre-work, or with people who don’t know much and need a lot, or for people somewhere in between. Assigning the same pre-work toevery personwill servenone of themadequately. We’d be far better off assessing each individual and offering a range of options based on specific needs. But for whatever reason, that’s almost never done. We get stuck on the “average need” and cater to it exclusively.
In both of these cases, the problem is that we use summary statistics like the average even though probably we shouldn’t be summarizing at all. If we are interested in accommodating every member of the population, what business do we havebasing anything onsummary statistics anyway? The entire point of many summary statistics is that they hide variation and complexity – they hide the mess, if you will. But sometimes the mess is exactly what we need to see and deal with. Which is counter-intuitive, and counter to a lot of the training we Six Sigma folks give and receive.
(For some related thoughts on this topic, see Holly Hawkins last blog entry. Standardization is not always the answer!)