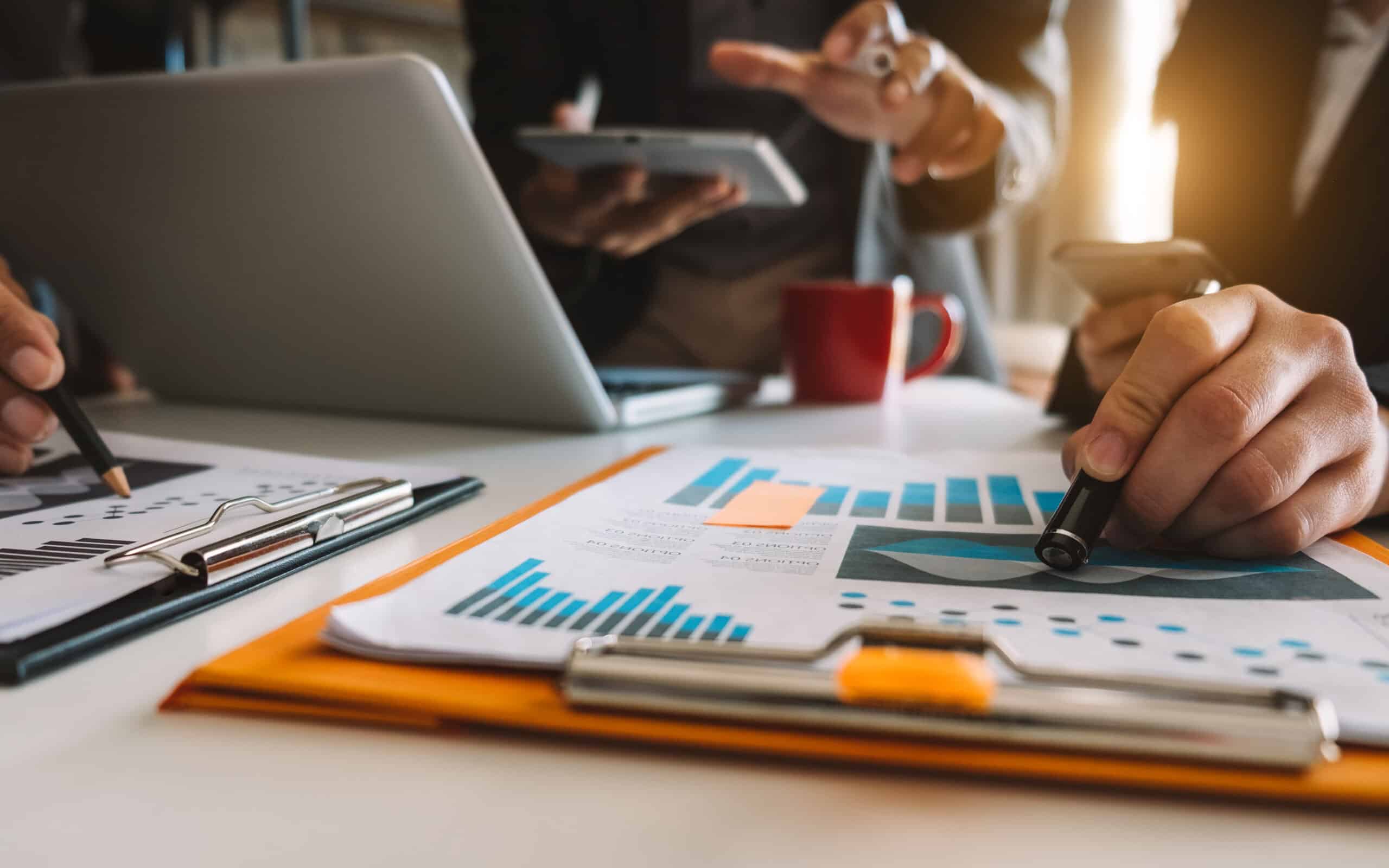
You will see the term alpha risk, or alpha error, come up when you are talking about hypothesis testing.
Hypothesis testing is a branch of statistics in which, using data from a sample, an inference is made about a population parameter or a population probability distribution. Risk can be defined as the probability or chance of making an incorrect decision.
This article will discuss what is meant when we talk about an “alpha risk,” its implications, and how to minimize the impact of making an alpha error.
Overview: What is an alpha risk?
Before you can fully understand what we mean by alpha risk, you should have a little background in hypothesis testing.
In hypothesis testing, you will state some assumptions about your data, and then go about trying to prove whether they are true or not. In all cases, the first thing you do is state the null and alternate or alternative hypotheses.
The word null in the context of hypothesis testing means “nothing” or “zero.”
For example, your null hypothesis might be that there is no difference in average sales between your current marketing plan and your new marketing plan. Your alternate hypothesis would say that there is a difference.
Since you’re using sample data to make your inferences about the population, there is a risk that you’ll make an error. In the case of the null hypothesis, you have a risk of making one of two errors.
- Alpha error, also known as a type 1 error or producer error: An alpha error is when you mistakenly reject the null and believe that something significant happened. In other words, you announced that your marketing program worked, when it didn’t.
- Beta error, also known as a type 3 error, or consumer error: A beta error is when you fail to reject the null when you should have. In this case, your marketing program worked, but you missed it and failed to take action.
A classic example is when you get the results back from your doctor after taking a blood test. As you know, there’s always a risk of giving you the wrong information because of testing errors. If the doctor says you have an infection when you really don’t, that’s an alpha error. The implications are that you have to spend money on antibiotics and may need to have more tests.
An alpha error is thinking there is something significant going on when there isn’t. We also call that a false positive. The doctor rejected the null that “there was zero infection” and missed the call.
On the other hand, if the doctor told you that everything was OK when you really did have an infection, then she made a beta, or type 2, error. Implications here are that you continue to feel sick without any relief. She failed to reject the null hypothesis when she should have. That is called a false negative.
Alpha is the risk you are willing to assume of falsely rejecting the null. The good news is that you decide on the level of your alpha risk. The typical values for alpha are 1%, 5%, or 10%. In other words, depending on the importance of the conclusion, you only want to falsely claim a difference or change when there isn’t one 1%, 5%, or 10% of the time.
The decision whether to reject your null is guided by something called the p-value, which is calculated from the data. The p-value is the actual risk you have in being wrong if you reject the null. You would like that to be low.
Your decision as to what to do about the null is made by comparing the alpha value (your assumed risk) with the p-value (actual risk). If the actual risk is lower than your assumed risk, you can feel comfortable in rejecting the null and claiming something has happened. But, if the actual risk is higher than your assumed risk, you will be taking a bigger risk than you want by rejecting the null.
3 ways understanding alpha risk can help your processes
The stating and testing of the null hypothesis is the foundation of hypothesis testing. Your alpha risk will give you a sense of how much error you’re willing to make when deciding what to do about your null hypothesis.
1. You get to choose your level of risk
It’s assumed that there will be risk whenever you’re making decisions based on sampled data. You’re able to choose the level of risk you’re willing to accept for falsely rejecting the null hypothesis.
2. You can choose your risk ahead of time
You should choose your alpha risk prior to doing any testing or data collection. Adjusting your acceptance criteria after you have analyzed your data is not recommended.
3. It’s easy to decide on next actions
By comparing your chosen alpha risk to the calculated p-value, you will usually have a clear decision for next steps with respect to your null hypothesis. When the p-value and alpha risk are very close, you may have to consider your decision a little bit more carefully.
Why is alpha risk important to understand?
Since your alpha risk is a measure of the chance you have of making a misjudgement or error, it’s pretty important that you understand what it means and how to minimize your chance of error.
Looking foolish
If you select an inappropriate alpha risk and falsely claim success for something when, in fact, it was a big dud, you may look foolish in front of your boss. This will not help your career.
Confidence
Confidence is defined as 1 minus alpha. For example, if you chose an alpha risk of 5%, you can have 95% (100% – 5%) confidence in your decisions. The concept of confidence may help guide you to an appropriate level of alpha risk.
P-value
It is the comparison of your chosen risk (alpha) to the calculated risk (p-value) that helps drive your decision whether to reject or not reject your null hypothesis. You need to understand the differences in the two risks.
An industry example of using alpha risk
In the pharmaceutical industry, it’s common to test new drugs in phases using relatively small sample sizes. One of the key metrics is whether there is a difference in the side effects between the new drug and a placebo. It’s hoped that there is no difference and patients will not suffer any significant side effects of the new drug.
A large pharmaceutical company was doing testing on a new drug that was designed to mitigate the symptoms of a common disease state. As was common, they used a small sample during the early stages of testing. They were only willing to assume a low alpha risk, so they chose 1%.
After collecting some of the initial data, the p-value was high (6%), so they didn’t reject their null hypothesis, which stated that there was no difference in side effects. They perceived that as a good thing and moved the drug to the next and much more expensive phase.
Again, testing showed that there was no significant difference in side effects, although the difference between the p-value and alpha risk was smaller. Again they were pleased and moved the product along — and finally launched it. As is protocol in the industry, data continued to be collected post-launch. Now they were collecting very large data sets.
Unfortunately, the p-value is sensitive to sample sizes, and as the data set grows, the p-value will go down. After many months of continued data collection, the p-value suggested that there was a statistically significant difference in the occurrence of side effects, with the new drug having more. The company had to address that issue publicly with the FDA and ended up having to reformulate the drug to try and reduce the undesired side effects.
3 best practices when thinking about alpha risk
Using hypothesis testing to help make better data-driven decisions requires that you properly address the null hypothesis. Your alpha risk helps you with that decision.
1. Select your alpha risk at the beginning
You either have a level of risk you’re willing to assume or you don’t. Selecting your alpha risk after seeing the results is gaming the system. Set your alpha risk at the beginning, and let your decision fall where it may based on the test results.
2. If in doubt, be flexible
There are situations where your alpha risk may be very close in value to your p-value. Your alpha risk may be 5% and your p-value 4.5%. According to the common practice of rejecting or not rejecting the null, you would reject the null if your calculated risk (p-value) is lower than your assumed risk (alpha). But with values that close, what should you do? Be flexible and don’t be dogmatic about the guidelines for rejecting the null. Take another look at your data.
3. Pick a reasonable alpha risk so you’re not always failing to reject the null hypothesis
Being too cautious will lead you to make beta errors, and you’ll never learn anything about your population data.
Frequently Asked Questions (FAQ) about alpha risk
What’s the relationship between alpha and beta risk?
Alpha is the risk of falsely rejecting the null hypothesis, while beta is the risk of failing to reject the null hypothesis when you should.
What’s an alpha error?
An alpha error, or Type 1 error, is rejecting the null hypothesis and claiming a significant event has occurred when, in fact, that is not true, and the null should not have been rejected.
How do I use the alpha error and p-value to know what decision I should make about the null hypothesis?
The most common way of answering this is: If the p-value is low (less than the alpha), the null should be rejected. If the p-value is high (greater than the alpha) then the null should not be rejected.
Becoming familiar with alpha risk
Alpha risk is the chance of error you are willing to make by rejecting the null hypothesis when you shouldn’t have. In most business applications, this error will result in financial impact.
For example, you did some test marketing on a new product, and based on your sample results, you conclude that the product will be a big success. Your null hypothesis was that the product will not be successful, and your alternative hypothesis was that it would be successful. Your p-value suggested that you could reject your null based on your alpha risk of 5%. Unfortunately, you were wrong, and the new product was a bust. You only discovered that after a national rollout.
That probably cost the company a lot of money — but hopefully it didn’t cost you your job!