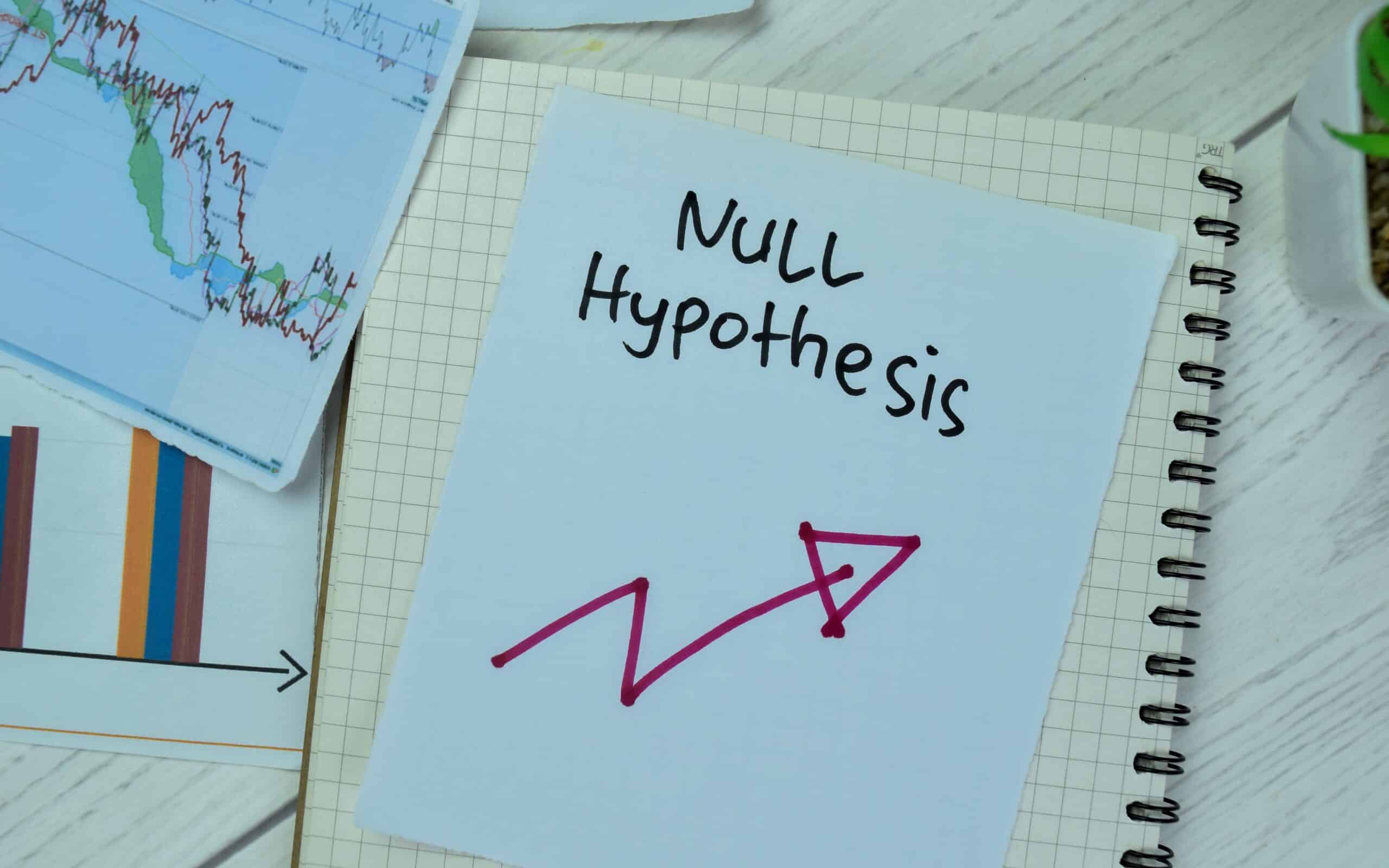
Hypothesis testing is a branch of statistics in which, using data from a sample, an inference is made about a population parameter or a population probability distribution.
First, a hypothesis statement and assumption is made about the population parameter or probability distribution. This initial statement is called the Null Hypothesis and is denoted by Ho. An alternative or alternate hypothesis (denoted Ha), is then stated which will be the opposite of the Null Hypothesis.
The hypothesis testing process and analysis involves using sample data to determine whether or not you can be statistically confident that you can reject or fail to reject the Ho. If the Ho is rejected, the statistical conclusion is that the alternative or alternate hypothesis Ha is true.
Overview: What is the Null Hypothesis (Ho)?
Hypothesis testing applies to all forms of statistical inquiry. For example, it can be used to determine whether there are differences between population parameters or an understanding about slopes of regression lines or equality of probability distributions.
In all cases, the first thing you do is state the Null and Alternate Hypotheses. The word Null in the context of hypothesis testing means “nothing” or “zero.”
As an example, if we wanted to test whether there was a difference in two population means based on the calculations from two samples, we would state the Null Hypothesis in the form of:
Ho: mu1 = mu2 or mu1- mu2 = 0
In other words, there is no difference, or the difference is zero. Note that the notation is in the form of a population parameter, not a sample statistic.
Since you are using sample data to make your inferences about the population, it’s possible you’ll make an error. In the case of the Null Hypothesis, we can make one of two errors.
- Type 1, or alpha error: An alpha error is when you mistakenly reject the Null and believe that something significant happened. In other words, you believe that the means of the two populations are different when they aren’t.
- Type 2, or beta error: A beta error is when you fail to reject the null when you should have. In this case, you missed something significant and failed to take action.
A classic example is when you get the results back from your doctor after taking a blood test. If the doctor says you have an infection when you really don’t, that is an alpha error. That is thinking that there is something significant going on when there isn’t. We also call that a false positive. The doctor rejected the null that “there was zero infection” and missed the call.
On the other hand, if the doctor told you that everything was OK when you really did have an infection, then he made a beta, or type 2, error. He failed to reject the Null Hypothesis when he should have. That is called a false negative.
The decision to reject or not to reject the Null Hypothesis is based on three numbers.
- Alpha, which you get to choose. Alpha is the risk you are willing to assume of falsely rejecting the Null. The typical values for alpha are 1%, 5%, or 10%. Depending on the importance of the conclusion, you only want to falsely claim a difference when there is none, 1%, 5%, or 10% of the time.
- Beta, which is typically 20%. This means you’re willing to be wrong 20% of the time in failing to reject the null when you should have.
- P-value, which is calculated from the data. The p-value is the actual risk you have in being wrong if you reject the null. You would like that to be low.
Your decision as to what to do about the null is made by comparing the alpha value (your assumed risk) with the p-value (actual risk). If the actual risk is lower than your assumed risk, you can feel comfortable in rejecting the null and claiming something has happened. But, if the actual risk is higher than your assumed risk you will be taking a bigger risk than you want by rejecting the null.
RELATED: NULL VS. ALTERNATIVE HYPOTHESIS
3 benefits of the Null Hypothesis
The stating and testing of the null hypothesis is the foundation of hypothesis testing. By doing so, you set the parameters for your statistical inference.
1. Statistical assurance of determining differences between population parameters
Just looking at the mathematical difference between the means of two samples and making a decision is woefully inadequate. By statistically testing the null hypothesis, you will have more confidence in any inferences you want to make about populations based on your samples.
2. Statistically based estimation of the probability of a population distribution
Many statistical tests require assumptions of specific distributions. Many of these tests assume that the population follows the normal distribution. If it doesn’t, the test may be invalid.
3. Assess the strength of your conclusions as to what to do with the null hypothesis
Hypothesis testing calculations will provide some relative strength to your decisions as to whether you reject or fail to reject the null hypothesis.
Why is the Null Hypothesis important to understand?
The interpretation of the statistics relative to the null hypothesis is what’s important.
1. Properly write the null hypothesis to properly capture what you are seeking to prove
The null is always written in the same format. That is, the lack of difference or some other condition. The alternative hypothesis can be written in three formats depending on what you want to prove.
2. Frame your statement and select an appropriate alpha risk
You don’t want to place too big of a hurdle or burden on your decision-making relative to action on the null hypothesis by selecting an alpha value that is too high or too low.
3. There are decision errors when deciding on how to respond to the Null Hypothesis
Since your decision relative to rejecting or not rejecting the null is based on statistical calculations, it is important to understand how that decision works.
An industry example of using the Null Hypothesis
The new director of marketing just completed the rollout of a new marketing campaign targeting the Hispanic market. Early indications showed that the campaign was successful in increasing sales in the Hispanic market.
He came to that conclusion by comparing a sample of sales prior to the campaign and current sales after implementation of the campaign. He was anxious to proudly tell his boss how successful the campaign was. But, he decided to first check with his Lean Six Sigma Black Belt to see whether she agreed with his conclusion.
The Black Belt first asked the director his tolerance for risk of being wrong by telling the boss the campaign was successful when in fact, it wasn’t. That was the alpha value. The Director picked 5% since he was new and didn’t want to make a false claim so early in his career. He also picked 20% as his beta value.
When the Black Belt was done analyzing the data, she found out that the p-value was 15%. That meant if the director told the VP the campaign worked, there was a 15% chance he would be wrong and that the campaign probably needed some revising. Since he was only willing to be wrong 5% of the time, the decision was to not reject the null since his 5% assumed risk was less than the 15% actual risk.
3 best practices when thinking about the Null Hypothesis
Using hypothesis testing to help make better data-driven decisions requires that you properly address the Null Hypothesis.
1. Always use the proper nomenclature when stating the Null Hypothesis
The null will always be in the form of decisions regarding the population, not the sample.
2. The Null Hypothesis will always be written as the absence of some parameter or process characteristic
The writing of the Alternate Hypothesis can vary, so be sure you understand exactly what condition you are testing against.
3. Pick a reasonable alpha risk so you’re not always failing to reject the Null Hypothesis
Being too cautious will lead you to make beta errors, and you’ll never learn anything about your population data.
Frequently Asked Questions (FAQ) about the Null Hypothesis
What form should the Null Hypothesis be written in?
The Null Hypothesis should always be in the form of no difference or zero and always refer to the state of the population, not the sample.
What is an alpha error?
An alpha error, or Type 1 error, is rejecting the Null Hypothesis and claiming a significant event has occurred when, in fact, that is not true and the Null should not have been rejected.
How do I use the alpha error and p-value to decide on what decision I should make about the Null Hypothesis?
The most common way of answering this is, “If the p-value is low (less than the alpha), the Null should be rejected. If the p-value is high (greater than the alpha) then the Null should not be rejected.”
Becoming familiar with the Null Hypothesis (Ho)
The proper writing of the Null Hypothesis is the basis for applying hypothesis testing to help you make better data-driven decisions. The format of the Null will always be in the form of zero, or the non-existence of some condition. It will always refer to a population parameter and not the sample you use to do your hypothesis testing calculations.
Be aware of the two types of errors you can make when deciding on what to do with the Null. Select reasonable risks values for your alpha and beta risks. By comparing your alpha risk with the calculated risk computed from the data, you will have sufficient information to make a wise decision as to whether you should reject the Null Hypothesis or not.