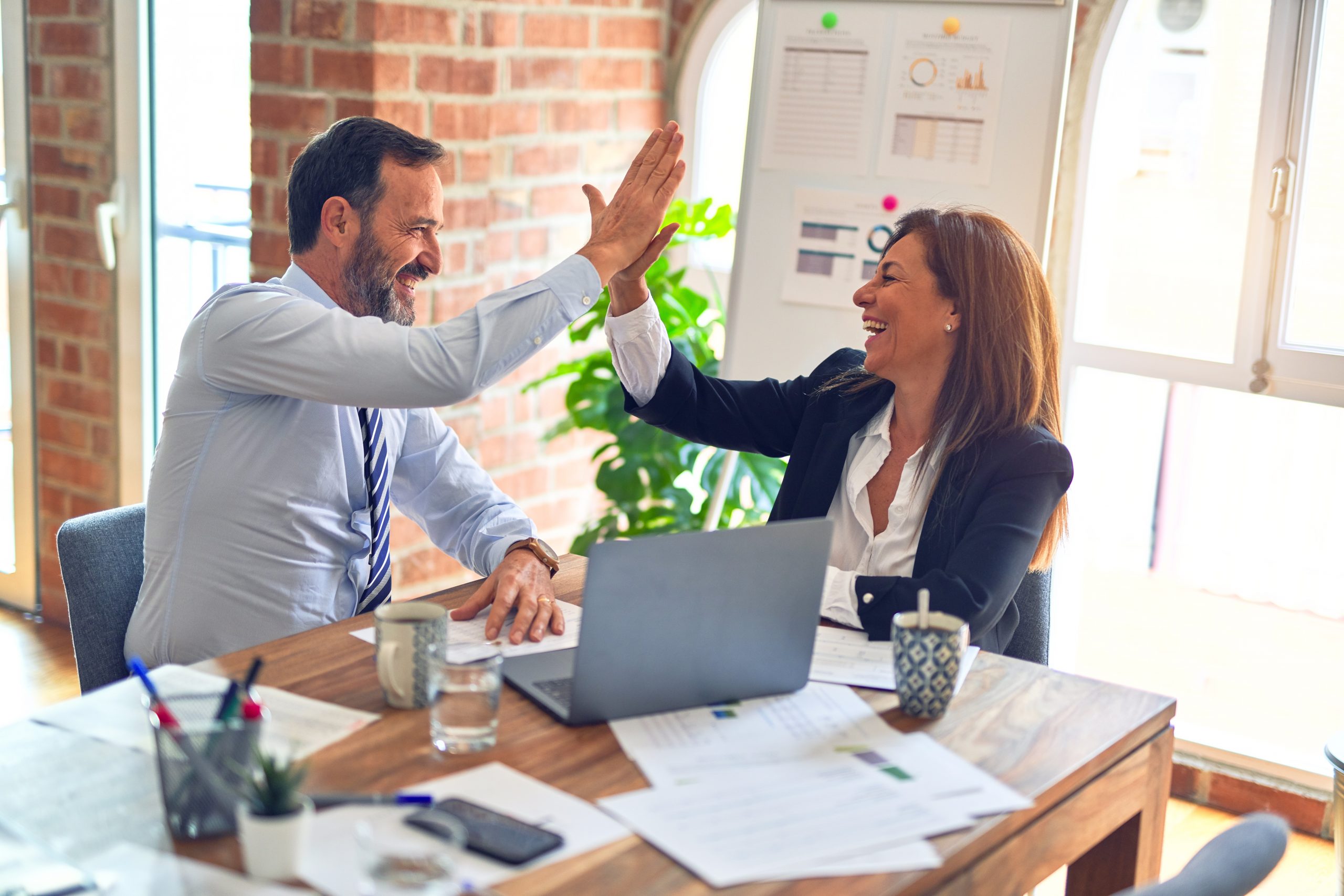
I have been working with my colleague, Dave Baker, here at Aviva and we have been researching the long-term behaviour of our processes.
Now I am not going to go down the path of process capability analysis, Cp, Cpk, Zshift and the like. Take a look at this simple time series plot to see why.
Over the long-term some of our processes are extremely seasonal and what we were interested inisunderstanding was how much of the process variation was due to seasonality, long-term trend or random process variation. It has been a bit of the blind leading the blind; we have reviewed statistics books, experimented in Minitab and tried to figure this out.
What we found was Minitab has a capability to decompose the data and with a bit of experimentation we produced an output that showed the seasonal variation and long-term trend.
From this we could eyeball when the process (black-line) moved away from the seasonally expected value (red-line) and also the long-term trend. We then found how to strip-out the seasonal-variation to see the process performance as shown below.
So we believe we have discovered the basics for long-term trendanalysis and some of the benefits appear to be:
- Ability to see degree to which seasonality affects process performance
- Ability to better understand long-term trends
- Gaina clearer picture of process performance without seasonal interference
- Ability to address short-term customer issues that can be explained by seasonality.
We have some way to go yet but feel we are moving in the right direction and this is getting us a lot of success on a current project.