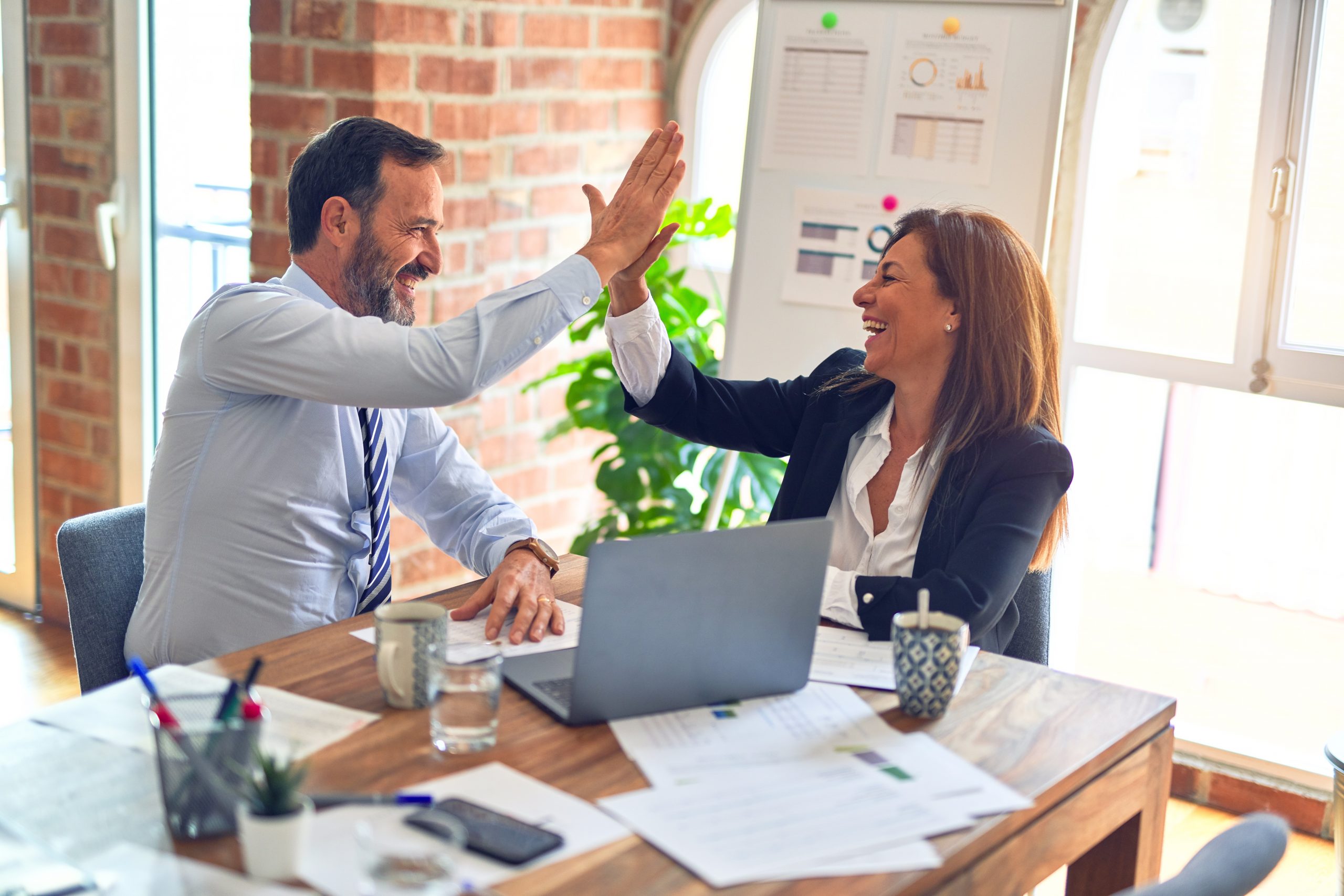
iSixSigma released a process sigma calculator which allows the operator to input process opportunities and defects and easily calculate the process sigma to determine how close (or far) a process is from 6 sigma. One of the caveats written in fine print refers to the calculator using a default process shift of 1.5 sigma. From an earlier poll, greater than 50 percent of polled quality professionals indicated that they are not aware of why a process may shift 1.5 sigma. My goal is to explain it here.
I am not going to bore you with the hard core statistics. Every Green, Black and Master Black Belt learns the calculation process in class. If you did not go to class (or you forgot!), the table of the standard normal distribution is used in calculating the process sigma. Most of these tables, however, end at a z value of about 3. In 1992, Motorola published a book (see chapter 6) entitled Six Sigma Producibility Analysis and Process Characterization, written by Mikel J. Harry and J. Ronald Lawson. In it is one of the only tables showing the standard normal distribution table out to a z value of 6.
Using this table you’ll find that 6 sigma actually translates to about 2 defects per billion opportunities, and 3.4 defects per million opportunities, which we normally define as 6 sigma, really corresponds to a sigma value of 4.5. Where does this 1.5 sigma difference come from? Motorola has determined, through years of process and data collection, that processes vary and drift over time – what they call the Long-Term Dynamic Mean Variation. This variation typically falls between 1.4 and 1.6.
After a process has been improved using the Six Sigma DMAIC methodology, we calculate the process standard deviation and sigma value. These are considered to be short-term values because the data only contains common cause variation — DMAIC projects and the associated collection of process data occur over a period of months, rather than years. Long-term data, on the other hand, contains common cause variation and special (or assignable) cause variation. Because short-term data does not contain this special cause variation, it will typically be of a higher process capability than the long-term data. This difference is the 1.5 sigma shift. Given adequate process data, you can determine the factor most appropriate for your process.
In Six Sigma, The Breakthrough Management Strategy Revolutionizing The World’s Top Corporations, Harry and Schroeder write:
“By offsetting normal distribution by a 1.5 standard deviation on either side, the adjustment takes into account what happens to every process over many cycles of manufacturing… Simply put, accommodating shift and drift is our ‘fudge factor,’ or a way to allow for unexpected errors or movement over time. Using 1.5 sigma as a standard deviation gives us a strong advantage in improving quality not only in industrial process and designs, but in commercial processes as well. It allows us to design products and services that are relatively impervious, or ‘robust,’ to natural, unavoidable sources of variation in processes, components, and materials.”
Statistical Take Away: The reporting convention of Six Sigma requires the process capability to be reported in short-term sigma – without the presence of special cause variation. Long-term sigma is determined by subtracting 1.5 sigma from our short-term sigma calculation to account for the process shift that is known to occur over time.