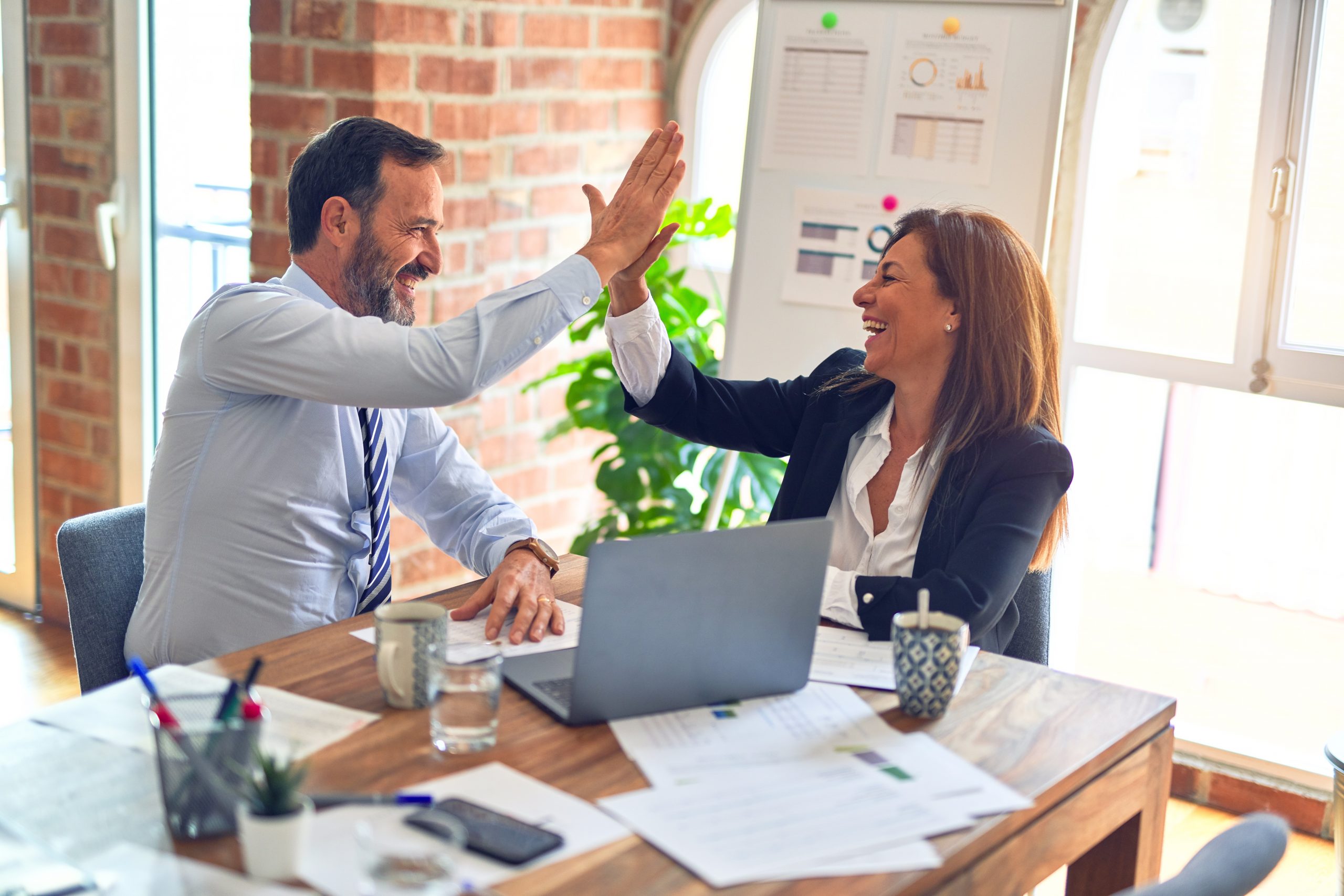
Suppose we consider a specific bilateral critical-to-quality characteristic (CTQ). In other words, we are in view of a key design feature that sports a two-sided performance specification. If a bilateral design margin of “Y percent” was to be imposed on the given CTQ (during the course of product configuration), we indirectly and necessarily infer the need for a level of capability that corresponds to a certain Z value (i.e., sigma level). Of course, this assumes a normal density function and that “unity” is prescribed by the +/- 3s limits of the corresponding distribution.
Unsurprisingly, the theoretical nature of such a performance construct implies an infinite degrees-of-freedom. However, the qualification of a process is usually initiated and conducted with far fewer degrees-of-freedom. In fact, many capability studies are based on the general range 30 < n < 250. Given this, we must continually acknowledge and operate in the presence of random sampling error when conducting capability studies and improvement activities. This is also true when assigning the performance expectations (i.e., tolerances) to the various CTQs associated with a product or service design. Much too often, the design engineers (and process engineers) do not know how to properly intersect the need for design margin (performance guard banding) with the need for process capability, especially in the presence of random sampling error.
Given this argument, we naturally seek a level of “pre-qualified capability” that anticipates or otherwise accounts for such error before-the-fact – often in the context of “worst-case conditions.” In other words, we seek to employ a design-process qualification procedure that can effectively and efficiently compensate for the assumed presence of “worst-case” random sampling error during the course of process qualification. Currently, there is a general dearth of available literature on how this should be done.
In concert with this line of reasoning, we must consider the cost-of-poor-quality. When doing so, we intuitively recognize that its relative magnitude is highly dependent upon the “quality of marriage” between a product’s many performance specifications and their corresponding process capabilities. From a different angle, we assuredly recognize the need to maintain the integrity of this relationship, regardless of whether or not sampling error is present during the course of process qualification. Thus, we have the primary aim of “robust design.”
The process capabilities associated with a given design must ultimately, adequately, sufficiently, and efficiently be “formulated and fitted” to the performance requirements – in a robust manner. Of course, the inverse of this is also true. Usually, the idea of “good design” involves the use of both approaches. Knowing “when to use what” is often the secret sause, so to speak. If we cannot realize this aim, then the idea of being able to “design in quality” will remain a dream in most organizations.
Here is the challenge (should you choose to accept it) – define a valid, widely applicable statistical methodology that satisfies the aforementioned problem (from a process as well design engineering perspective). For more details on the nature of this problem (and the architecture of an existing solution), reference the forthcoming book entitled “Resolving the Mysteries of Six Sigma: Statistical Constructs and Engineering Rationale” by Mikel J. Harry, Ph.D.