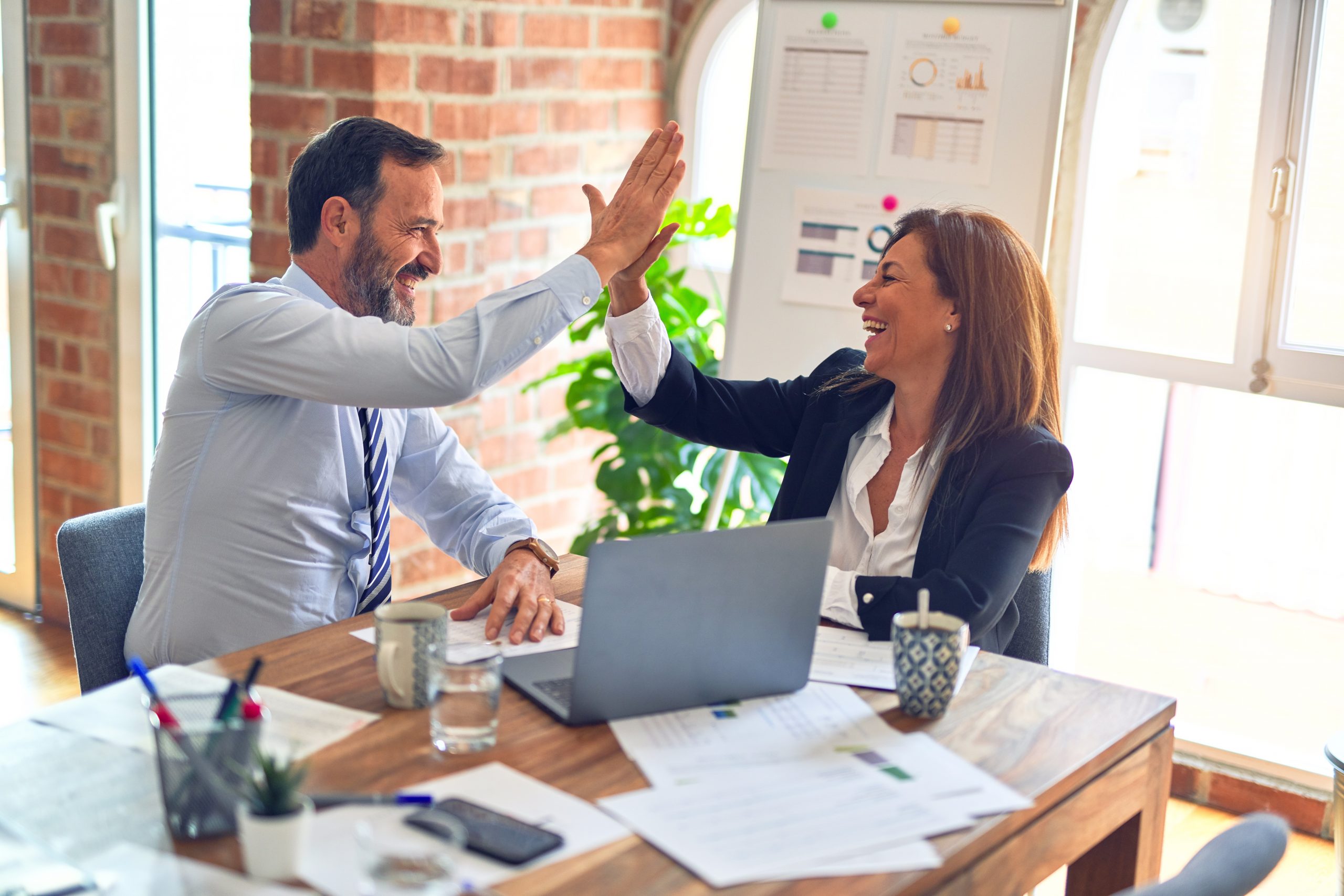
Six Sigma is the buzzword of today. Companies big and small talk about it and numerous success stories further advertise its relevance in today’s challenging business environment. Debates on its emergence as a strategic initiative have created critics who consider it old wine in a new bottle and loyal followers willing to swear by it.
The latter of these are practitioners who view Six Sigma as an effective way to implement statistical thinking, a philosophy of learning and action based on the following fundamental principles:
- All work occurs in a system of interconnected processes
- Variation exists in all processes
- Understanding and reducing variation are the keys to success
Statistical thinking provides practitioners with the means to view processes holistically. Table 1 reflects the Six Sigma tools that map to these principles, which are not exhaustive in nature and could overlap with other principles.
Table 1: Six Sigma Tools And Principles | |
Principle | Six Sigma Tools |
All work occurs in a system of interconnected processes | Process Maps, Value Stream Mapping, Input and Output Process Diagrams, SIPOC, Cause and Effect Matrix, Failure Mode Effects Analysis (FMEA), Quality Function Deployment (QFD) |
Variation exists in all processes | Descriptive Statistics, Measurement System Analysis (MSA), Enumerative Statistics, Multi-Vari Charts, Cause and Effect Diagrams, Box Plots, Process Capability Analysis |
Understanding and reducing variation are the keys to success | Analytic Statistics, Control Charts, Design of Experiments (DOE), Hypothesis Testing, Correlation, Regression Analysis, Pareto Charts |
Many Black Belts feel compelled to apply Six Sigma tools in the order learned during training. For example, using Control Charts, taught in the Measure Phase, before Design of Experiment tools, which are taught in the Improve Phase. Black Belts can often complete projects faster and obtain better results using statistical thinking practices rather then DMAIC as their only guideline. To illustrate this point, I would like to share two case studies where different approaches were used, both employing statistical thinking principles.
Case Study 1
Shafts were ground on machines to a close tolerance of +- 5 Microns, representing a process capability of less than 1.0. The objective of the project was to increase the Cpk to 1.5. Using conventional DMAIC as a guideline, the team created a detailed process map. The map was created after a comprehensive study of the machine manual, which also allowed identification of the critical Xs. A Failure Mode Effects Analysis (FMEA) was then completed to identify risks associated with each critical X and a Design of Experiment (DOE) was conducted to optimize speed, feed, coolant and other controllable parameters. As a result of the team’s effort, the grinding process was optimized and a Cpk of 1.68 achieved. Control charts were used to document the effectiveness of shaft monitoring and a Control Plan was established to control the critical Xs.
In this Case Study the first principle of statistical thinking, all work occurs in a system of interconnected processes, was employed during the initial phase of the project. Process Capability Analysis and Process Mapping were key elements of this phase. During the second phase of the project, use of Control Charts and a FMEA emphasized the use of the second principle, that variation exists in all processes. The success of the project, illustrated through the use of DOE and Control Charts, was achieved by understanding and reducing variation, the third statistical thinking principle.
If the Black Belt first implemented a DOE without studying the machine manual and employing Control Charts, noise experienced during experimentation would lead to inconclusive results. The Black Belt would reach the conclusion that Control Charts should be analyzed prior to attempting a DOE and that the only variation in the process is due to common causes.
Case Study 2
In a credit card processing organization the response time between swiping a credit card to approval or decline is critical. The objective of the project was to improve the process such that no response would take more than 14 seconds from the moment of dialing to response. The team first mapped the network to gain an understanding of the process flow then used the conventional DMAIC methodology. Data was collected and analyzed through Multi Vari Charts. At this stage of the project, the team felt that they did not understand much of the variation and, instead of continuing to employ the conventional DMAIC methodology, elected to implement a DOE. At the end of the experiment, 92 percent of the variation control factors impacting response time were identified. This finding greatly assisted the team in transitioning from the Measure phase to Control phase in just two days!
The following review of advantages and disadvantages can help determine whether to apply DOE or Control Charts first.
Applying DOE First To Understand Variation
Advantages:
- Gives conceptual understanding of the process early in the project, leading to quick completion of the project.
- Helps understand the cause-and-effect relationship early in the project.
- Helps identify the components for the Y= f (X) equation earlier in the project.
- Encourages a sequential approach for DOE.
Disadvantages:
- Does not typically distinguish between special causes and common causes. If there is a special cause during experimentation it will appear in a residual analysis of the experiment.
- Too much noise during experimentation may hide significant Xs.
- A lack of understanding of how special causes impact the process may create a difficult environment for sustaining results after experimentation.
- Process understanding of special causes may remain poor.
Applying Control Charts First To Understand Variation
Applying control charts first to understand variation is a more conventional approach followed by most Six Sigma projects.
Advantages:
- Helps understand the variation of the process and eliminate Special Causes that can affect the process thus eliminating undue noise during future applications of DOE.
- Enables an understanding of whether the variation is time dependent and helps gain an understanding of appropriate factors for Blocking in experimentation.
- Helps analyze the process dynamically and provides a means for obtaining quick feedback to rectify process drift.
Disadvantages:
- Creates time constraints for solving problems. Effective charts contain a minimum of 25 sub-groups, which can lead to long data collection periods.
- Hinders identification of direct Cause and Effect relationships and creates environment where Special Causes are difficult to distinguish.
In summary, I would like to emphasize that the best results are achieved when statistical thinking principles are used as a guideline. The above case studies illustrate that there is no set rule to understanding variation based on the order of the tools used. Black Belts should review each project independently to determine the most effective use of these tools and look to utilize them judiciously rather than with a standard cookbook approach.
Reference
ASQ Statistical Division, Glossary of Statistical Terms. (2003). Available On-line: http://www.asq.org.