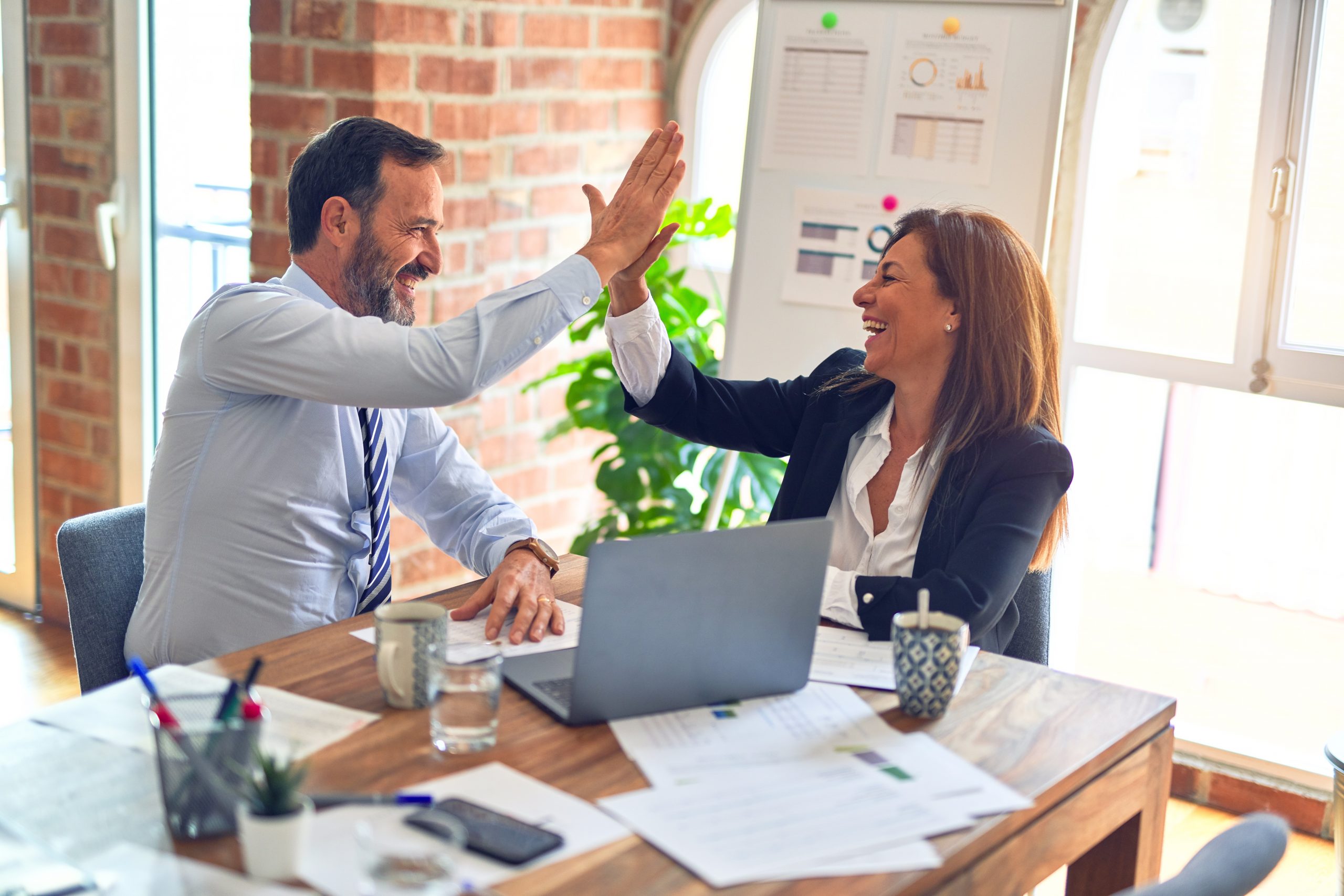
In my on-going journey to master the tools & techniques of Lean Sigma I have been reviewing the materials on ANOVA. How useful this has been, it has really moved me on a level.
ANOVA comes across in training as a very useful tool and Tukey’s pairwise comparison really hits the spot. However in our business with samples of skewed non-normal distributions with unequal variance, I infrequently meet the assumptions for use, “Samples must be normally distributed and of equal variance” and swap over to Mood’s Median Test.
So when I reviewed this topic in my own time I was surprised to read in Principles of Applied Statistics that “in practise the normality and equal variance assumptions are not important” I was intrigued. Not having a statistical background I had always assumed these were fixed assumptions and used the tools accordingly. Could it be that I had misunderstood the theory? What about the other statistical tests based on the normal distribution e.g. 1-sample t-test, 2-sample t-test, paired t-test and F-test for equal variance, could these be used as well?
I started doing some empirical tests in Minitab. Useful research but did not answer the questions. I then reread my collection of Six Sigma books. These gave differing and sometimes vague advice on how to handle hypothesis tests on non-normal & unequal variance samples. From this I got two things, first I needed to look outside of my “Universe” to find an information source I could trust, second I needed to be more specific in my questions. So I framed the questions:
- To what degree does non-normality impact the hypothesis test?
- To what degree does unequal variance impact the hypothesis test?
To find an information source I could trust I went to the library and borrowed the biggest academic book on statistics I could find, Introduction to the Practice of Statistics. It went in-depth in the areas I was looking for guidance.
In terms of output I wanted to produce a simple table of practical advice that could be used. Here is what it looks like now, still working on it and going through the theory.
HypothesisTest | Non-normal Data | Unequal Variance |
1-sample t-test | Robust test except against outliers or strongly skewed data. Samplesless than 15 – must have normal data,less than 40 -no outliers or strongly skewed andgreater than40 – no outliers but can be skewed | No significant impact |
2-sample t-test |
In research | In research |
Paired t-test | In research | In research |
F-test | Do not run test, use Levene’s test | In research |
ANOVA | In research | Largest standard deviation should be less than twice smallest standard deviation |
This is the start of the research and joins the growing body of knowledge I am building in the subject. What it does is provoke the next set of questions, things like what is the risk of using the results, how does sample size impact on confidence intervals in these situations, what other situations should be considered e.g. outliers & different distributions and when should non-parametric tests be used.
Anyone out there with the answers?