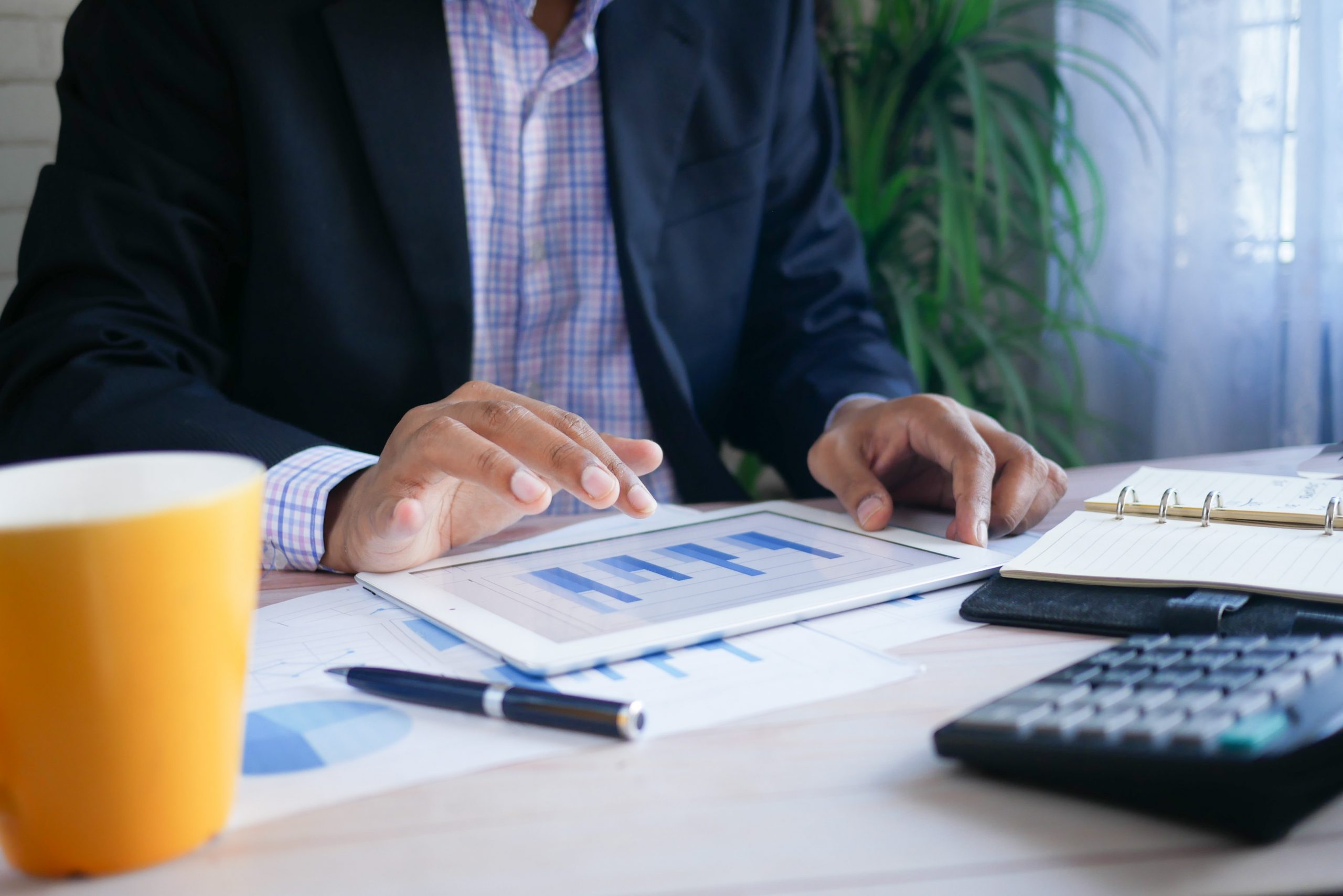
What Is Linearity?
Before we can understand linearity, we must understand another measurement error known as bias. Bias is a consistent mistake that occurs in measurement. For example, every measure taken with your scale comes out heavier than the actual weight.
Linearity measures the consistency of a bias over the entire range of possible measurements. Returning to the weight example used above, if your scale weighs heavier than the actual weight, is that difference a consistent one pound over no matter how heavy the object might be? Or does the variation from actual weight increase as the weight increases?
The Benefits of Quantifying Linearity
There are three main benefits of determining the linearity of your measurements:
1. Lets You Know How Bias Changes Over An Entire Range
Knowing how a bias changes over the entire measurement range is a critical piece of information if you wish to limit the impact of measurement error on your process studies.
2. Helps You Determine How Much Variation Is Due to Measurement Error
It’s hard to determine the cause of output differences in a study unless you know how much of that variation is due to measurement error. If the variation found in a study is no more than the expected measurement error, then the difference isn’t statistically significant.
3. Discover Quality of Measurement System
How good is your measurement system? Can you rely on it, or do you need better equipment or measurement methods? Performing a linearity study can help engineers and managers answer these questions and eliminate sources of measurement error.
How to Calculate Linearity
Calculating linearity requires the following eight steps:
1. Take at least five samples spread across the entire range of possible measurements.
2. Find the reference value for each sample (determine what the actual value should be).
3. Have the operator measure each sample at least ten times.
4. Calculate bias for each measurement (measured value minus reference value).
5. Average the bias for each part.
6. Plot the average bias for each part on a chart.
7. Find the “best fit” line.
8. Interpret results.
If the best fit line is horizontal, then the linearity is consistent. The larger the slope of the best fit line, the more linearity varies as the measurement changes.
What is Repeatability?
Repeatability is the variation that occurs when one operator measures the same part multiple times.
The Benefits of Finding Repeatability
The three primary benefits of determining repeatability are:
It Can Help You Establish the Accuracy of a Gauge or Tool.
There are two possible variation sources when measuring a part. Either the variation is due to the part you measure, or it comes from the measurement tool. Under ideal circumstances, any variation comes from the part, not the measurement method.
Repeatability Is Usually Easy to Find
Determining repeatability is usually a simple process. All you need to do is have the same operator measure the same part multiple times.
Helps Analysts Find Problems In Measurement Systems
If the same operator measuring the same part multiple times leads to a lot of variation, there is likely a problem with your measurement process. The operator, measurement method, or tool you’re using isn’t adequate for the job.
Large variation shows that there is a problem. Analysts must study the measurement process to determine the cause.
How to Calculate Repeatability
Calculating repeatability requires the same operator to measure the same part multiple times and record the data. You can then measure the possible range of values by picking out the high and low measurements from the data. Finally, analysts can calculate the average variation by adding up the total variance and dividing by the number of measurements.
Linearity Vs. Repeatability
As mentioned above, the main difference between linearity and repeatability is the number of parts measured. Linearity involves measurements of multiple parts, often taken by different operators, and can include various measurement tools. A linearity study aims to determine if a measurement bias exists and to find how that bias varies over the possible range of measurements. A linearity study also involves comparing these measurements to a known reference.
Conversely, repeatability requires the same operator to measure the same part using the same tool. Here the goal is to find the expected variation using a particular device. There is no comparison of that measurement to a known reference value.
Who Would Use Linearity and Repeatability?
Both repeatability and linearity studies will be used by analysts concerned with finding and characterizing measurement errors in a process. In a linearity study, analysts assume any variance caused by the operators is minimal, and the main driver of any difference comes from the process or tools used to measure the parts. A linearity study aims to discover how a measurement process handles different parts.
On the other hand, a repeatability study attempts to “tease out” data variance inherent in measuring a single part. While both concepts involve discovering measurement errors, they focus on different types of errors.
Choosing Between Linearity and Repeatability: Real World Scenarios
Suppose an engineering team notices that support beams produced by a particular process show too much variance in weight. In this situation, they might order a repeatability study to determine the source of this difference in weight. If the beams produced by certain operators show high levels of weight variance, then the solution might be to retrain those operators or dismiss them. On the other hand, if all operators produce beams with wildly varying weights, then the source of the error is either the scale used to make the measurements or the production process.
However, if the engineering team suspects that measurement errors are concentrated in either particularly heavy or light support beams, they might order a linearity study. In this case, the team will want to know if any measurement bias exists and if that bias remains consistent over the entire range of beams produced by the process. If heavy beams show significant variations from the actual weight, the solution might be to use a different measurement method for heavier beams. If all beams show a consistent bias over the entire range, then the answer might be to make a simple data adjustment to compensate for the bias.
In some situations, analysts could use linearity and repeatability studies concurrently. For example, suppose a linearity study shows a consistent measurement bias. In that case, the analyst team could use a repeatability study to estimate how much of the weight differences they’re seeing are due to bias and how much is variance inherent in their measurement method.
Linearity and Repeatability Seek To Eliminate Different Kinds of Measurement Errors
Both linearity and repeatability studies seek to limit measurement mistakes. However, linearity focuses on errors caused by measuring different parts, while repeatability concentrates on mistakes inherent in the measurement process itself.