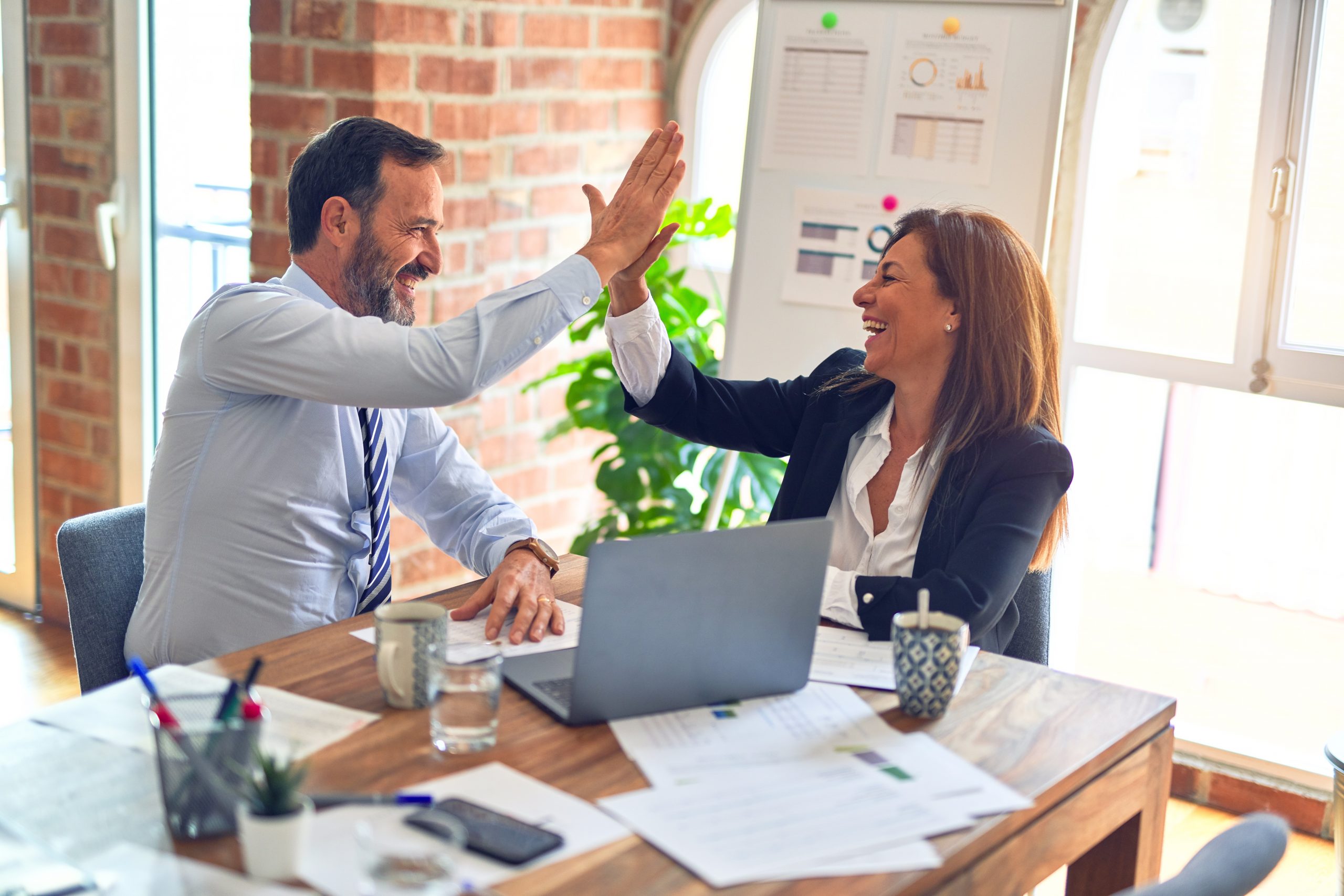
When performing statistical data analyses, quality professionals are always challenged to maintain data integrity. When should you round up the answer; when should you round down? How many significant figures are appropriate for the data set that has been taken?
Below are a set of simple rules that should help you traverse the perils of statistical data analysis.
Variation Data Round-off
Rule: Round off the answer to one more significant figure than present in the original data.
Rule: This rule is only valid for final results, not intermediate values.
Example: Cycle-time data for an application receipt to account opening process is as follows (in days): 4, 4, 3, 5, 1, 5. The mean of these values is 3.66666666…, and should be rounded to 3.7. Because the original data were whole numbers, we round the answer to the nearest tenth.
Probability Data Round-off
Rule: Either provide the exact fraction or decimal of the probability or round-off the final result to three significant digits.
Example: The probability of rolling a ‘4’ with a single die is 1/6 or 0.16666666…, which would be rounded-off to 0.167. The probability of a coin landing on ‘tails’ is 1/2 or 0.5 – because 0.5 is an exact figure, it is not necessary to express it as 0.500.
Sample Size Round-off
Rule: When the calculated sample size is not a whole number, it should be rounded up to the next higher whole number.
Rule: Rounding up a sample size calculation for conservativeness ensures that your sample size will always be representative of the population.
Example: A sample size calculation determined that 2006.083 data points were necessary to represent the population. In this case, 2007 data points samples should be taken.