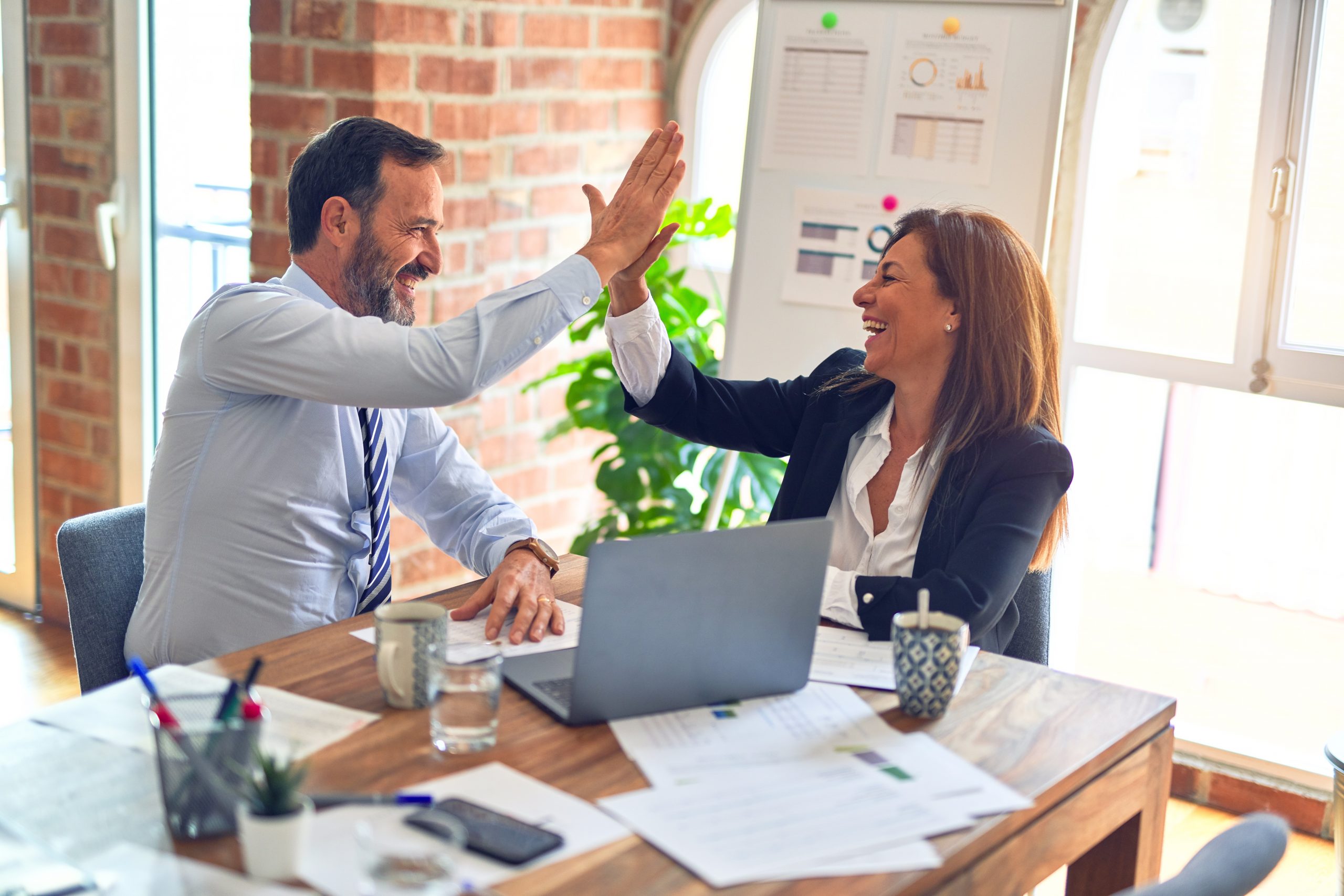
Many companies spend considerable amounts of money on customer surveys every year. They then use those survey results to amend strategies, design new products and services, focus improvement activities and to celebrate success. But can practitioners always rely on the results they see?
Here is a fictional example: MyInsurance, a life insurance company with worldwide market reach, was celebrating the success of improving its customers’ satisfaction in 2006. The company proudly presented these results: “In Thailand we have achieved 58 percent satisfied customers as compared to 2005, when it was only 54 percent.” This sounds good, right? In a market with millions of consumers, an increase in satisfaction of 4 percent would mean the number of customers who would happily buy from MyInsurance again has increased by some 10,000.
But this conclusion could be wrong. For obvious reasons, MyInsurance did not ask millions of customers for their opinions. They gathered opinions from 280 customers. This approach is called sampling and is being applied in every kind of company many times a day.
Understanding Sampling
When companies sample, they gather data from a comparatively small number of customers to draw conclusions about the population, which is the entire pool of customers in whose opinions a company is interested. Sampling has a huge advantage: It saves money and time, and is especially useful when the process of testing can destroy the object, such as drop testing of mobile phones. This advantage is paid for with a disadvantage: the margin of error, or confidence interval.
Determining the Confidence Interval
The confidence interval is the range in which a practitioner expects the population value to be. In sampling, it is only possible to guess what the “real” value is. This confidence interval cannot be avoided, even with a perfectly representative sample under ideal conditions. Practitioners can improve the interval, however, by increasing the sample size and by decreasing the variance in the population. The latter usually is not possible. Hence, a practitioner’s only choice is to determine the minimum sample size for the confidence interval they are expecting.
In the case of MyInsurance, using a 95 percent confidence level, it is possible to determine that in 2005, the “real” customer satisfaction level was between 48 percent and 60 percent. In 2006, it was between 52 percent and 64 percent. The risk for assuming the company’s customer satisfaction has improved is 35 percent.
If MyInsurance wishes to distinguish between a customer satisfaction level of 54 percent and 58 percent, it needs to have confidence intervals for each of those percentages that do not overlap. Hence, confidence intervals of +/- 2 percent are needed for at least one, or both at best.
Based on the estimation of the sample size for this requirement, MyInsurance would need to involve nearly 2,500 customers in its satisfaction survey each year. From the sample of 280 customers they have taken, it is likely that there has been no change, or worse, a decrease in customer satisfaction. It is impossible to know without more data to give a better result.
Gain a Better Understanding of Sampling with this Experiment
Buy one 200g package of M&M’s and count the number of pieces in the package. This number is the population. Now count the number of yellow M&M’s. In one instance, this experiment resulted in a population of 233, with 43 yellow pieces, meaning the population is 18.5 percent yellow.
Sampling means taking a small number of M&M’s out of the population in a representative way. For example, in a bowl full of M&M’s, pulling 20 out blindly resulted in no yellow pieces. Putting those 20 back into the population and counting a new sample of 20 revealed 4 yellow M&M’s. Eight more samples resulted in 2, 3, 3, 6, 3, 5, 4 and 3 yellow M&M’s, respectively.
Doing the math, those samples suggested that the population has 0 percent, 20 percent, 10 percent, 15 percent, 15 percent, 30 percent, 15 percent, 25 percent and 15 percent yellow, respectively. Which sample is correct? None. All of the samples give only an indication for the real percentage of yellow in the population.
Sampling results vary even though the population is untouched. Drawing conclusions based on this variation may result in expensive mistakes.
Shifting Data Viewpoints
Often, important decisions are based on a small sample of data that is poorly collected. Many practitioners do not determine the confidence data carries. They emphasize the average, which is easy to calculate and easy to understand. But every mean coming out of a sample is only correct for that sample, not the population that the practitioners are trying to make a decision about.
Management would benefit in decision making by changing the way they look at data and following this advice:
- Do not trust means.
- Ask for the confidence interval.
- Avoid making decisions based on small samples.