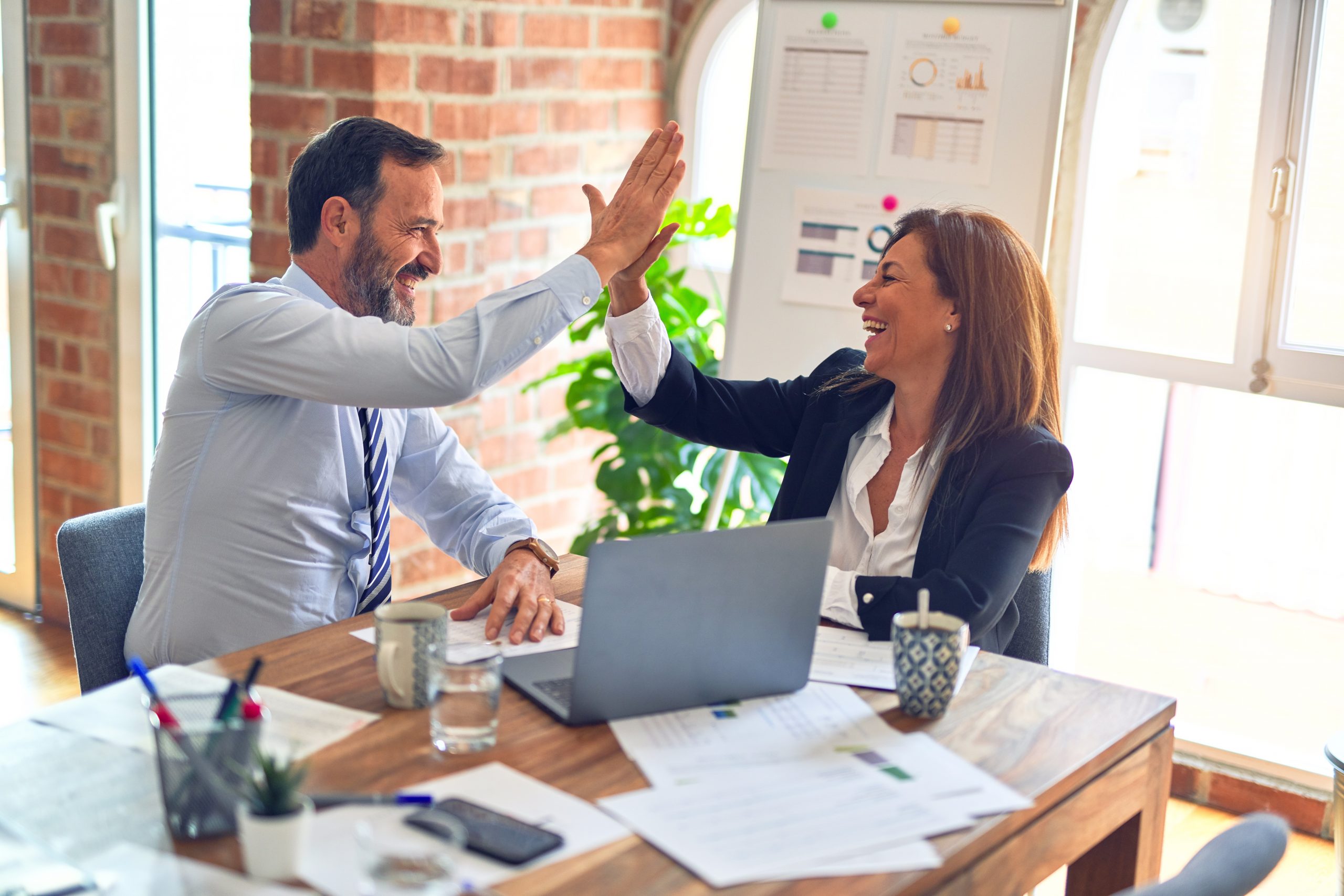
Most quality professionals recognize that Six Sigma is a breakthrough strategy. They understand that the methodology uses data to measure current process effectiveness and to validate improvement; uses proven statistical and quality tools to identify process gaps and improvement solutions; and uses change management processes for institutionalizing and integrating improvements in an organizations’ operational and business strategy.
Still, it is curious that so many do not understand how Six Sigma can be used in the information technology industry to improve processes and produce bottom-line benefits. Here, a case study offers an example of how a production support team used Six Sigma to reduce the number of IT requests, issues and tickets initiated by end-users by 70 percent.
Isolating the Business Opportunity
As part of the production support project, the team:
- Extracted/received data files from various source systems.
- Transformed the data as per business requirement.
- Loaded transformed data into the data warehouse.
- Generated various reports for business users for data analysis and decision making.
- Resolved issues raised by business users within the service-level-agreement period.
Challenges surfaced when it was observed that the work day was being extended beyond the normal working timing to resolve issues, and pending ticket count on any day was around 90. Upon investigation it was found that the average number of issues raised in a month was 120. And that number required rework efforts of 1,000 person-hours. This was significantly more than the 500 person-hours for which the company had planned. This contributed to a reduction in profit margin for this “fixed cost” project. The project team set a target to reduce the rate of ticket generation by 50 percent.
Analyzing the Problems
The project team examined all the tickets raised during the previous three months. Based on the ticket closure history, all the tickets were divided into four basic categories – performance issues, data issues, enhancement requests and administrative requests. A Pareto analysis revealed that 70 percent of tickets were from the data issue category. Further analysis, using a data flow diagram (see figure below), compiled from a detailed process map of load monitoring process and cause-and-effect diagram, identified all possible potential causes for data issues. The team then used an impact-control matrix to evaluate each cause for its impact, feasibility and control. It helped team to come out with the vital few causes for data issues in the data warehouse.
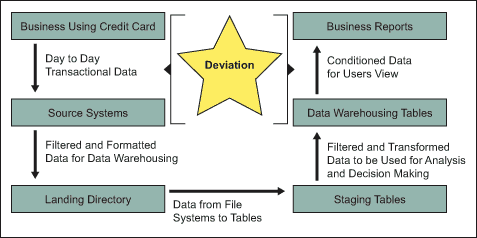
Some of the root causes identified were:
- Wrong logic for data filter and transformation.
- Missing or duplicate data in the data warehouse.
- Record rejection in the extract-transform-load process.
- Data missing in the input file.
- Improper recovery and restart of load during interim load failure.
- Missing loads.
Finding Solutions for Root Causes
Structured brainstorming was conducted among the project team members to identify various solutions for each root cause. The team decided to implement the following solutions:
- Automating the process of checking the input file layout correctness and data completeness.
- Identifying and fixing the extract-transform-load logic.
- Analyzing the rejected records during the loading process and reloading them.
- Auditing interim data in various critical tables of the data warehouse.
- Using a checklist for tracking the loading process.
- Preparing and using guidelines for the load recovery process in case of interim load failure.
- Defining and using critical data validation processes to check data accuracy in the data warehouse before releasing it to users.
The team identified risks involved with each solutions and respective mitigation plan by using failure mode and effects analysis. All the actions items were implemented with an appropriate change control mechanism.
Significant Results Achieved
On implementation of these proposed solutions, there was a 70 percent reduction in the number of tickets raised by business users. After data was collected for a three-month period, the average number of tickets opened in a month was 30, compared to 120 before improvement. For any given day, the new outstanding ticket count was reduced from 90 to 5. This reduced the workload in the team and the corresponding team size. This resulted in a positive impact on profits and data quality in data warehousing; and it helped customers (business users) in making value-added business decisions. This team established a benchmark for other data warehousing teams in successfully adopting the Six Sigma methodology for process improvement and profit optimization.