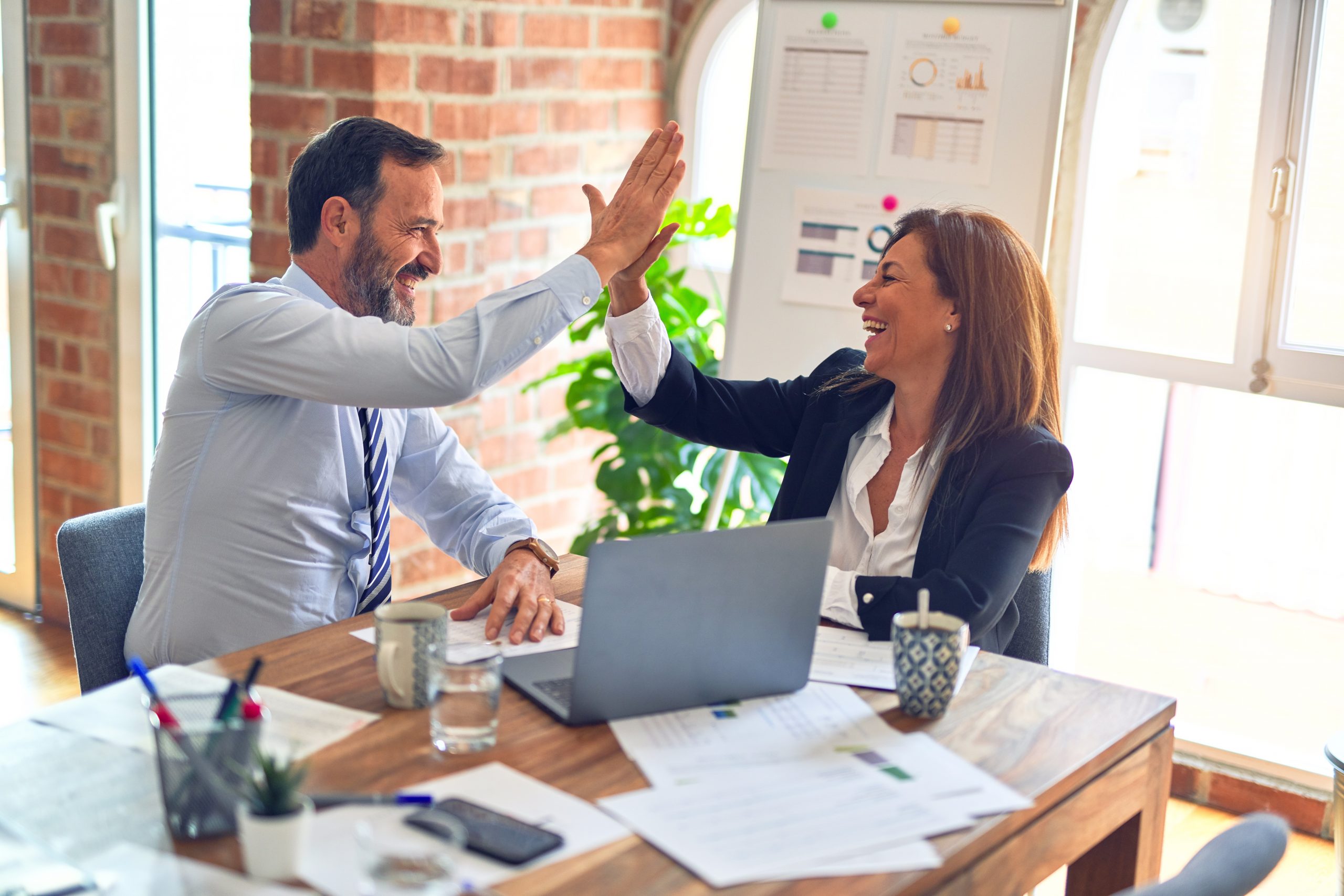
I recently was reminded of the value of challenging long-held assumptions. I was working in a 700-seat call center, which was operated by an international mobile carrier that had been purchased and merged with a large regional carrier. Systems and processes at the call center were finally stabilizing, except for the billing process. The company ran 19 billing cycles, shipping call records offshore for processing and development of the bill files, which were then returned to the United States for printing and mailing. It was not unusual for computer glitches to cause billing delays of up to several days. This resulted in large batches of work being dropped as the billing team scrambled to catch up.
My boss believed that the uneven release of bills was driving volume spikes into the call center. The issue at hand was to create a case for smoothing out the work stoppages caused by billing delays. Many in the call center thought this back-office function could be corrected over time by giving priority to other, more important features and functions under development. My boss sought immediate efforts to correct this basic service, and she had various analysts attempt to demonstrate the impacts.
Day-Over-Day vs. Week-Over-Week
Early attempts at proving correlation between delays in the release of bills and volume spikes at the call center used the assumption that bills arrived in the home an average of three days after being mailed. Analysts then compared the call volume three days after the bill mailings and found that the correlation (R value) was close to zero. Because the two volumes moved independently, the analysts proclaimed that call volume was not correlated to bill mailings.
Once I was asked to take a crack at the problem, I did not examine the math, but rather the assumptions used in the analysis (the math is almost always right). My hypothesis was that all contact with a consumer drives response – some contact is desirable (sales efforts), some is undesirable (unnecessary confusion interpreting bills) – so there should be an effect to bills being bunched up and released en masse. In marketing, a 1 to 2 percent response is normal and 5 percent is extraordinary. There was a risk that the effect of the bills would be so light that normal variation (sales promotions and customer needs, such as refilling pre-paid accounts) would mask the bill call volume shifts. Thus, the question, “Is there a correlation between bill drops and volume three days later?” was flawed. Using PDCA (plan-do-check-act) analysis, I took another turn at developing a new hypothesis.
The approach for analysis becomes obvious once you put yourself in the envelope with the bill and picture yourself being delivered to the customer. The mail did not always arrive in three days (variability), and most people don’t open the bill and call on day one. (This approach was the mental equivalent of the Lean principle “go and see.”) These variations mean that responses to arriving bills would not be immediate and would occur over time. I selected a week, rather than a specific day, as the metric that was most suitable for this analysis.
By comparing the week’s volume of bills mailed to the next week’s volume of calls, I was able to demonstrate the effects of late or spiked bill drops on call volume. I did the analysis for the prior six months using only a 26-week run chart to demonstrate the direction of the movement in volumes. To validate cause and effect, I used the general principle that the odds of call volumes and bill mailings moving in the same direction were 50-50. In my analysis, the two variables followed the same pattern for more than seven weeks – a significant finding. I observed three anomalies in this pattern during in the 26 periods, all of which could be explained by mailings from the previous week being made inordinately late in the week. There may have been other causes that I was unable to detect, but there was enough evidence for causality that I felt confident pressing ahead.
Sharing the Results
During my final presentation of this data to my boss, I also met with the person responsible for planning staff and coverage for our call center and proposed the following, based on my findings:
- All consumer contact drives a response
- Each contact type may have a particular response curve
- It may be useful for call volume projection to develop a model that contains the response curves
- It is possible to layer the response curves to smooth out spikes and improve response times
My analysis of this problem was admittedly crude, but it could be refined to achieve daily granularity. By factoring in customer behavior and other uncontrollable variables, practitioners can add a new perspective on the issue of call volume and billing cycle correlation.