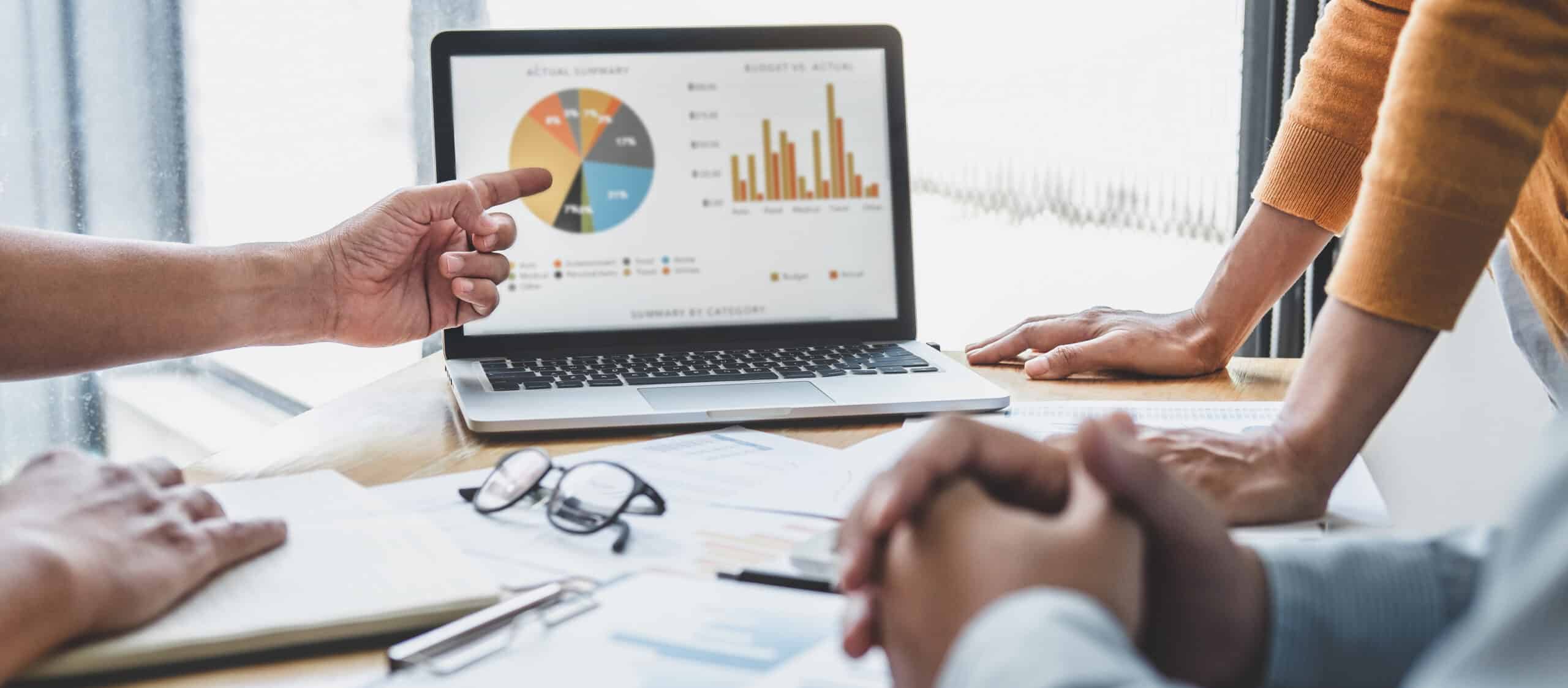
It is often not possible or practical to collect all the data from your process. It can be too costly or take too much time. It may not be possible to access all the data. If this situation exists in your process, then you will need to sample your data.
Overview: What is sampling?
There are two basic types of sampling. They are:
- Population – Drawing from a fixed group with definable boundaries. No time element.
- Process – Sampling from a changing flow of items moving through the business. Has a time element.
Which should you use?
- In process sampling, you measure characteristics of things or characteristics as they pass through the process and observe changes over time.
- Any data you collect that has time order included can be examined as either a population or a process – however, the size of the sample may need to be different.
- Given a choice, process data gives more information, such as trends and shifts of short duration. Process sampling techniques are the foundation of process monitoring and control.
Unfortunately, when you sample data, you can introduce bias. Here are some of the common types of sampling bias:
- Self-selection – choosing to opt into the sample
- Self-exclusion – choosing to opt out from the sample
- Missing key representatives – not including all relevant groups. For example, not including data from the third shift.
- Ignoring non-conformances – excluding data from the sample because it doesn’t feel right
The big pitfall in sampling is bias – i.e., select a sample that does NOT really represent the whole. Your sampling plan needs to guard against bias. Different methods of sampling have different advantages and disadvantages in managing bias.
Your sampling strategy should seek to minimize the within sample variation to maximize the chance of seeing between sample variation. This can be illustrated by the graphic below:
Here are the most common sampling strategies and whether they minimize the within sample variation.
- Random – used for population sampling but will not minimize within sample variation.
- Stratified random sampling – used for population sampling but will not minimize within sample variation.
- Randomly sample within a stratified category or group
- Sample sizes for each group are generally proportional to the relative size of the group
- This is recommended for doing population studies when you suspect that there is some uniqueness associated with a characteristic of the population (eg: gender)
- Systematic or periodic sampling – used for process or population sampling but will not minimize within sample variation.
- Sample every nth one (e.g., 4th one)
- Must select sampling frequency
- Watch out for bias in the frequency selected
- Suggested for process studies and possibly population
- Systematic and periodic sampling – will minimize within sample variation and best used for process sampling. Sample consecutive samples every nth time period.
This is how you would determine your minimum sample size for population or process sampling for continuous data:
For discrete data, you would use this formula:
3 benefits and 1 drawback of sampling
While it might be obvious that sampling has many benefits, let’s look at a few of them in more detail.
1. Saves time and money
By only collecting a subset of all your available data, you will be able to collect what you need in a shorter period of time. Additionally, by using a sample rather than all the data, it will be less expensive, especially if you are doing destructive testing of your samples.
2. Makes for easier data collection
By using one of the common strategies for collecting sample data, the task becomes easier and more flexible.
3. May be more accurate
When calculating your required sample size, you make the decision based on how accurate and precise you want your conclusions to be.
4. There will be increased uncertainty
Because you are taking only a subset, there will be a certain level of error in your conclusions. The good news is that you can determine what level of error is acceptable to you.
Why is sampling important to understand?
There are some important things to consider and understand when you are using sampling for your data collection. Here are a few:
Inherent error
Because you are using samples instead of the population of available data, there will be a certain level of sampling error.
Beware of bias
There is a natural tendency to introduce bias into your sampling scheme. Be aware it exists and set up protocols to reduce or eliminate this bias.
You must use the proper sampling strategy
There are several different sampling strategies depending on the nature of your process and why you are collecting data. Be sure to use the appropriate sampling strategy for what you are trying to learn about your process.
An industry example of sampling
A finance team wanted to know the minimum sample size required to collect data on the proportion of invoices that require rework after sending them to a customer. From interviews, the team concluded that approximately 25% of the invoices contained errors and required rework. They wish to determine the estimated % requiring rework within 5%. Below are their calculations:
3 best practices when thinking about sampling
Keep these hints in mind when doing your sampling otherwise your data and therefore your conclusions about the data may not be valid.
1. Do a MSA
Sampling invalid data will only lead to erroneous conclusions. By first using Measurement System Analysis (MSA) you will be comfortable that your data can be trusted. Sampling from trusted data will lead to correct conclusions about your process.
2. Determine the type of data you are sampling
Sample size calculations are based on the type of data you are sampling. There are different formulas for sampling continuous data and discrete data.
3. Determine the amount of sampling error you are willing to accept
When calculating the appropriate sample size, you will make the decision about how accurate (confidence interval) and how much precision (delta) you wish to have.
Frequently Asked Questions (FAQ) about sampling
What is the major downside of using sampling during data collection?
Once you start sampling your data rather than using it all, there will be a natural uncertainty or error associated with that sample. You can determine how much you are willing to accept as part of the sample size formula calculations.
What is the difference between random and stratified random sampling?
If you suspect there is a unique difference between groups in the population you are sampling, then you will want to use stratified random sampling. In this strategy, you randomly sample from the different groups in the proportion they exist in the population.
How is process sampling different from population sampling?
Population – Drawing from a fixed group with definable boundaries. There is no time element.
Process – Sampling from a changing flow of items moving through the process. This approach has a time element.
Summary
Sampling is a statistical technique for selecting a subset of samples for analysis. There are a number of issues to consider prior to collecting a sample from your data. Here is what you need to think about when doing sampling:
- What type of data do you intend to collect?
- Are you doing a process or population study?
- How do you plan on handling issues of bias?
- What is the most appropriate sampling strategy to use given what you wish to know from your sample?
- What is the minimum sample size you will need to accomplish your analytical goals?
Sampling will save you time and money but keep in mind that you will have some level of sampling error. Fortunately, you can select your desired level of accuracy and precision before you collect your sample.