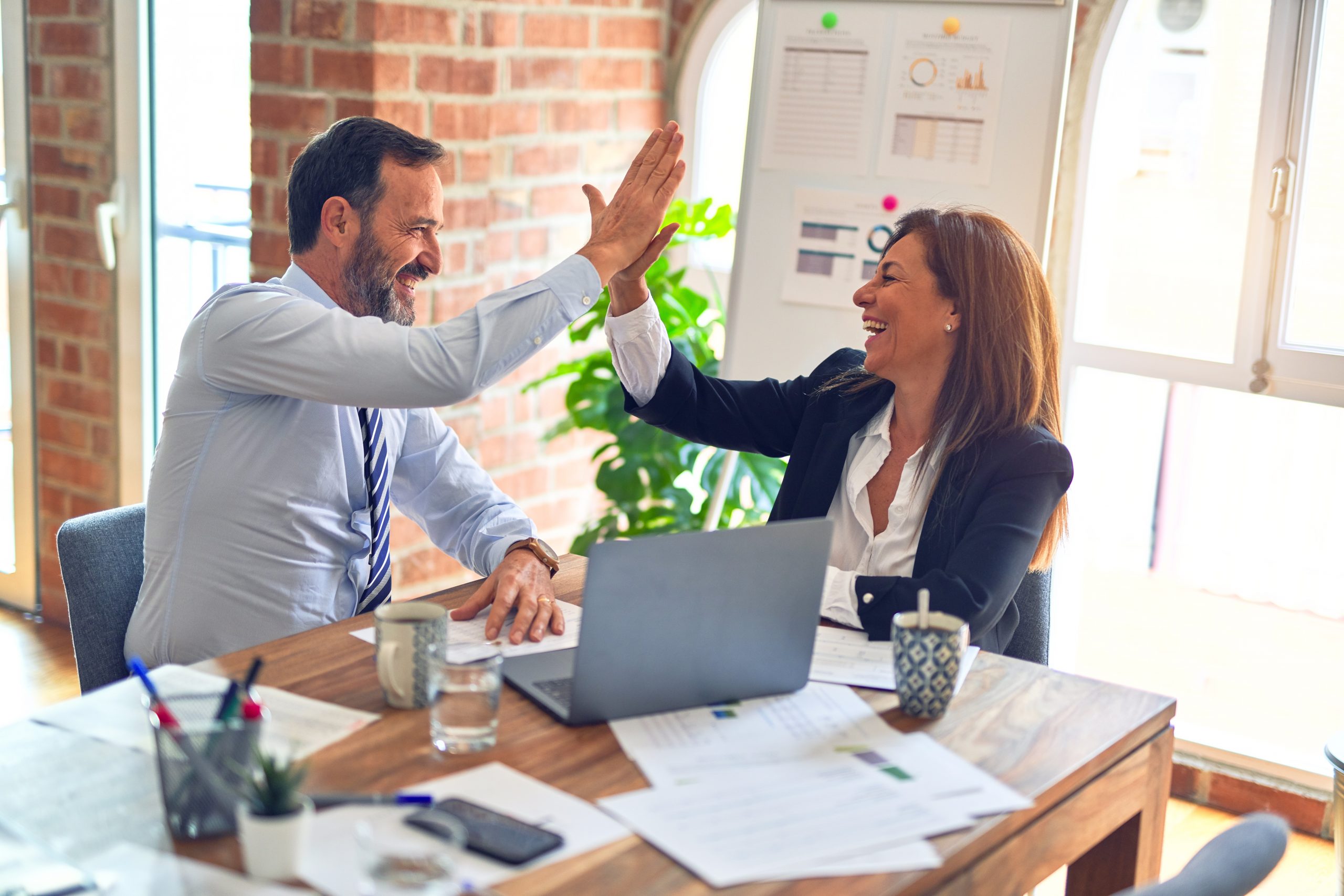
Most Master Black Belts would probably cite design of experiments (DOE) as the most underused statistical tool in financial services. Yet, designed experiments are a favorite tool of statisticians and Six Sigma practitioners working in general business environments because they are an extremely efficient way of identifying what matters in a process and what does not. That means businesses can focus their energies on the factors that really make a difference in effectiveness (quality, cost, customer satisfaction) and efficiency (speed).
What distinguishes design of experiments is that:
- Multiple factors are tested simultaneously for greatest efficiency. (Compare that to the traditional change-only-one-thing-at-a-time approach taught in most grade schools.)
- The results can pick up interactions between factors (how one factor influences another), something that is difficult to detect by other means.
- The experiment can provide a model of the process which can be used to conduct trade-off studies and reach an optimum solution.
The reason grade school science experiments were limited to only one variable at a time is because in those experiments there is no way to isolate the effects of one factor from those of another. DOE resolves that issue by using a particular kind of statistical analysis that allows differentiation among changes caused by different factors – even when all the factors are changed at the same time.
A typical designed experiment will involve anywhere from three to eight factors, each of which are tested in a specific combination at two settings or levels. (Some designs allow more factors and more levels, but these are used less frequently.)
Setting Up the Experiment
As an example of DOE in use, here is how a designed experiment helped a financial services company improve its application process. The bank was experiencing a 60 percent reprocessing (rework) rate on applications due to incomplete information provided by the customer. The project team trying to solve this processing problem suspected that three factors might affect whether an application was complete:
- The type of application
- How much detail was provided in the instructions
- Whether additional examples were provided
The team decided to study the application process in two locations, so in all there were five factors included in the experiment, each of which had two levels:
- A = Application Type: Loan versus lease
- B = Region: Midwest versus Northeast
- C = Description: Current versus enhanced (additional explanation provided)
- D = Example: Current versus enhanced (additional examples added)
- E = Negative Example: None versus yes (example added of what not to put in the blanks)
The experiment involved 16 trials, or runs, each with a different combination of factor settings. As shown in the table below, for example, the first run tested loan applications in the Midwest, with the current level of description and a negative example provided. The 12th run looked at lease applications in the Northeast with the current level of description, additional examples, but not negative examples. The response (y) measured by the team was the percent complete of each application. The table shows both the experiment design and the results (the “Avg. % Complete” column).
Run Order | Application Type | Region | Description | Example | Negative Example | Average % Complete | Standard Deviation
|
1 | Loan | Midwest | Current | Current | Yes | 46.5 | 8.4 |
2 | Lease | Midwest | Current | Current | None | 47.2 | 9.2 |
3 | Loan | Northeast | Current | Current | None | 40.5 | 5.9 |
4 | Lease | Northeast | Current | Current | Yes | 49.8 | 6.2 |
5 | Loan | Midwest | Enhanced | Current | None | 60.2 | 8.6 |
6 | Lease | Midwest | Enhanced | Current | Yes | 65.8 | 8.9 |
7 | Loan | Northeast | Enhanced | Current | Yes | 58.5 | 5.8 |
8 | Lease | Northeast | Enhanced | Current | None | 57.2 | 6.1 |
9 | Loan | Midwest | Current | Enhanced | None | 88.7 | 8.7 |
10 | Lease | Midwest | Current | Enhanced | Yes | 81.4 | 8.5 |
11 | Loan | Northeast | Current | Enhanced | Yes | 83.9 | 6.3 |
12 | Lease | Northeast | Current | Enhanced | None | 79.3 | 5.7 |
13 | Loan | Midwest | Enhanced | Enhanced | Yes | 91.6 | 8.4 |
14 | Lease | Midwest | Enhanced | Enhanced | None | 99.3 | 8.6 |
15 | Loan | Northeast | Enhanced | Enhanced | None | 96.3 | 5.8 |
16 | Lease | Northeast | Enhanced | Enhanced | Yes | 94.2 | 5.9 |
Results of the Experiment
Of the five factors originally studied, only two of them proved to influence the percent complete:
- Whether an example was provided (Factor D)
- How much description was provided (Factor C)
What did not matter was Region (the completion rate was the same in both the Northeast and Midwest); Application Type (the completion rate was the same for both loans and leases); and Negative Example (showing people what not to do did not affect completion rate).
These results are captured in Figure 1, where the steepness of the lines indicates the strength of the relationship. The lines for Description and Example change a lot, while the lines for the other three factors are nearly flat.Â
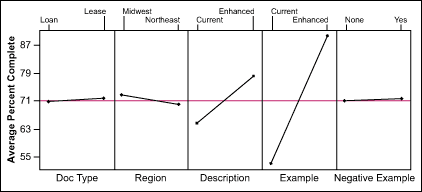
As noted above, the DOE also reveals interactions between factors – that is, whether one factor affects another. Look at Figure 2, for example. What it shows is that having a negative example made things worse in the Midwest but improved rates in the Northeast. (In statistical terms, this means there is an interaction between Negative Example and Region.)

From these results, the team concluded that for both types of applications:
- The same forms and process would be used in both regions, since the results were the same.
- It would help to provide enhanced descriptions and examples for certain fields.Â
By studying the results of the interactions closely, the team determined that negative examples did not help significantly when there were positive examples. And since the team had decided to use the positive examples, it would not pay to also develop negative examples.
The new forms increased the application completeness from 60 percent to more than 95 percent, virtually eliminating reprocessing and significantly reducing the cycle time for the loan and lease application process. The additional capacity that was made available as a result of the improved process allowed the business to generate additional revenue without increasing headcount.Â
Conclusion: Give DOE a Try
Many companies avoid using design of experiments in part because it sounds complicated. Actually, the example shows that the basic setup is straightforward. All that is needed is to select the factors to test and identify different levels for those factors. It is advisable to work with a Black Belt or Master Black Belt trained in DOE to help pick out the factors and levels. That expertise will make sure the company gets the most from its investment of time and effort. A Black Belt also can help analyze and interpret the results – a process which can go quickly with statistical analysis software that is readily available.
Most people who use DOE once become instant converts because the results usually have immediate practical application. It shows which process or design factors have the biggest impact on quality, speed, performance or delivery. That translates into better decisions.