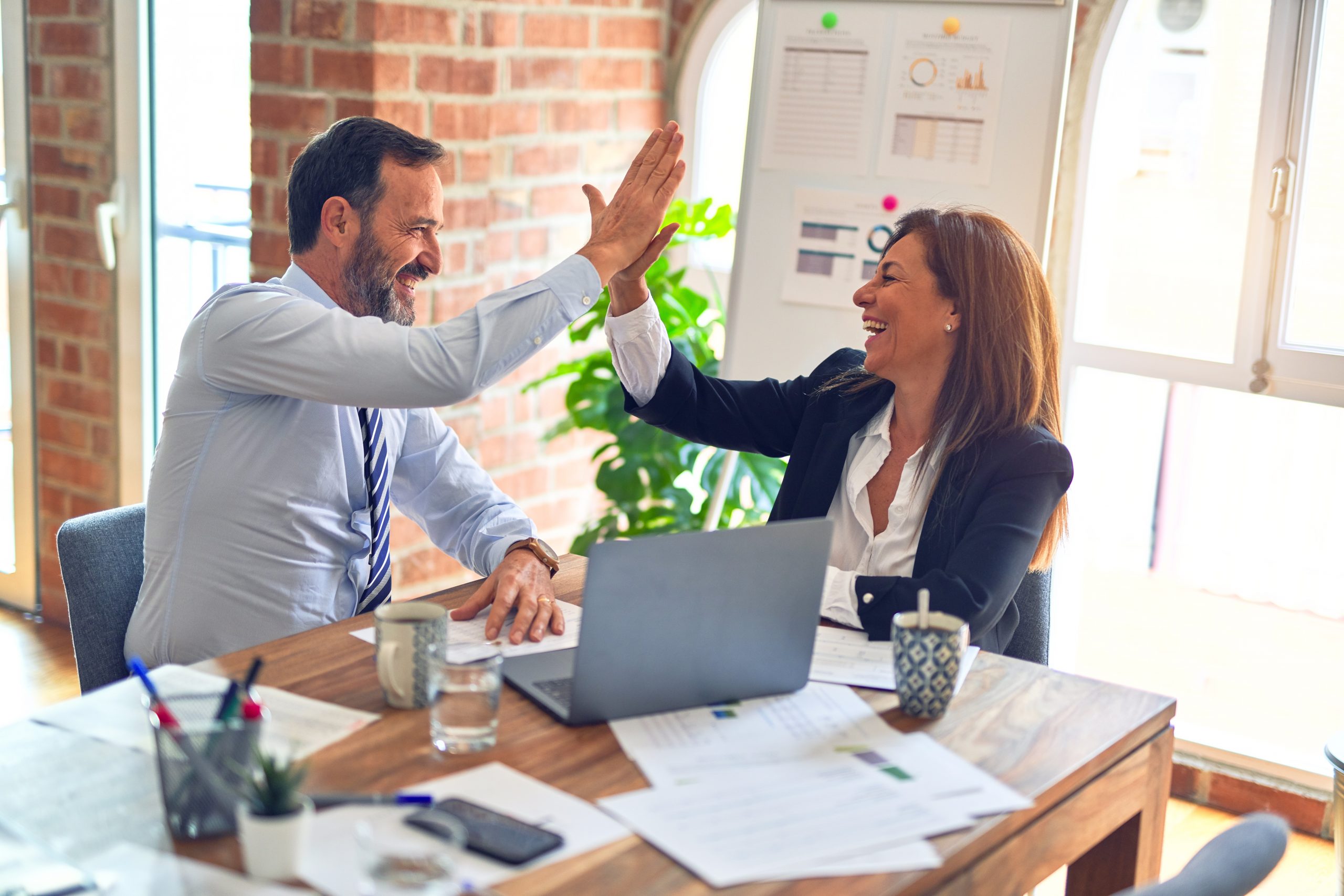
Black Belts and Six Sigma practitioners who are leading DMAIC (Define, Measure, Analyze, Improve, Control) projects should develop a sound data collection plan in order to gather data in the measurement phase. There are several crucial steps that need to be addressed to ensure that the data collection process and measurement systems are stable and reliable. Incorporating these steps into a data collection plan will improve the likelihood that the data and measurements can be used to support the ensuing analysis. What follows is a description of these steps. A checklist, populated with dummy responses, is also provided to illustrate the importance of building a well-defined data collection plan prior to execution.
Three phases – five steps total – are involved in building a sound data collection plan:
Pre-Data Collection Steps
1. Clearly define the goals and objectives of the data collection
2. Reach understanding and agreement on operational definitions and methodology for the data collection plan
3. Ensure data collection (and measurement) repeatability, reproducibility, accuracy and stability
During Collection Steps
4. Follow through with the data collection process
Post-Data Collection Steps
5. Follow through with the results
Step 1: Define Goals And Objectives
A good data collection plan should include:
- A brief description of the project
- The specific data that is needed
- The rationale for collecting the data
- What insight the data might provide (to a process being studied) and how it will help the improvement team
- What will be done with the data once it has been collected
Being clear on these elements will facilitate the accurate and efficient collection of data.
Step 2: Define Operational Definitions and Methodology
The improvement team should clearly define what data is to be collected and how. It should decide what is to be evaluated and determine how a numerical value will be assigned, so as to facilitate measurement. The team should consider consulting with the customer to see if they are already collecting the same (or similar) data. If so, comparisons can be made and best practices shared. The team should also formulate the scope of the data collection:
- How many observations are needed
- What time interval should be part of the study
- Whether past, present, and future data will be collected
- The methodologies that will be employed to record all the data
It is best to obtain complete understanding of and agreement on all the applicable definitions, procedures and guidelines that will be used in the collection of data. Overlooking this step can yield misleading results if members of the improvement team are interpreting loosely defined terms differently when collecting data. Serious problems can arise for the organization when business decisions are made based on this potentially unreliable data.
If the team wishes to examine historical data to include as part of the study, careful attention should be paid to how reliable the data and its source has been, and whether it is advisable to continue using such data. Data that proves to be suspect should be discarded.
Step 3: Ensuring Repeatability, Reproducibility, Accuracy and Stability
The data being collected (and measured) will be repeatable if the same operator is able to reach essentially the same outcome multiple times on one particular item with the same equipment. The data will be reproducible if all the operators who are measuring the same items with the same equipment are reaching essentially the same outcomes. In addition, the degree to which the measurement system is accurate will generally be the difference between an observed average measurement and the associated known standard value. The degree to which the measurement system is stable is generally expressed by the variation resulting from the same operator measuring the same item, with the same equipment, over an extended period.
Improvement teams need to be cognizant of all the possible factors that would cause reductions in repeatability, reproducibility, accuracy and stability – over any length of time – that in turn may render unreliable data. It is good practice to test, perhaps on a small scale, how the data collection and measurements will proceed. It should become apparent upon simulation what the possible factors are, and what could be done to mitigate the effects of the factors or to eliminate the factors altogether.
Step 4: The Data Collection Process
Once the data collection process has been planned and defined, it is best to follow through with the process from start to finish, ensuring that the plan is being executed consistently and accurately. Assuming the Black Belt or project lead has communicated to all the data collectors and participants what is to be collected and the rationale behind it, he or she might need to do additional preparation by reviewing with the team all the applicable definitions, procedures, and guidelines, etc., and checking for universal agreement. This could be followed up with some form of training or demonstration that will further enhance a common understanding of the data collection process as defined in the plan.
It is a good idea that the Black Belt or project lead be present at the commencement of data collection to provide some oversight. This way the participants will know right away whether or not the plan is being followed properly. Failure to oversee the process at its incipient stages might mean that a later-course correction will need to be made, and much of the data collection and/or measurement efforts will be wasted. Depending on the length of time it takes to collect data – and whether the data collection is ongoing – providing periodic oversight will help to ensure that there are no shortcuts taken and that any new participants are properly oriented with the process to preserve consistency.
Step 5: After The Data Collection Process
Referring back to the question of whether or not the data collection and measurement systems are reproducible, repeatable, accurate, and stable, the Black Belt or project lead should check to see that the results (data and measurements) are reasonable and that they meet the criteria. If the results are not meeting the criteria, then the Black Belt or project lead should determine where any breakdowns exist and what to do with any data and/or measurements that are suspect. Reviewing the operational definitions and methodology with the participants should help to clear up any misunderstandings or misinterpretations that may have caused the breakdowns.
Step 6: Sample Populated Data Collection Plan
The text displayed in maroon is example data for illustration purposes only. In order to create your own data collection plan, you should follow the outline provided and reproduce the maroon text with your project specific plan.
Goals And Objectives
Description of the project:
The results of the recent election in our municipality have caused concern over the validity of our vote counting process. Our current law states that a manual recount is required when the vote count differential is less than 0.5 percent. However, neither the manual vote counting process nor the vote counting device have been analyzed to determine their reliability. Such information will be beneficial to the legislature when they convene to discuss the state of our voting process. Therefore, the improvement team has decided to collect some data relating to the vote counting process. They will start the measurement phase with an experiment to determine if the punch-hole type ballots have any tendency to become altered or materially misshaped – such that the outcome (or vote) would change if the same ballot were subjected to a manual recount – as a result of being processed through the vote counting device. This one-factor-at-a-time experiment will explore the possibility that manual recounts, even if proven to be reliable, could give erroneous information if the ballots they receive (as inputs into the manual recount process) from the vote counting device have been altered in some way. Subsequent experiments will examine whether the practice of stacking and binding the punch-hole type ballots after they have been processed through the device would contribute to any alteration of outcomes.
Data to be collected:
Post-feed vote count accuracy.
Name of measure (label or identifier):
Vote count totals from pre-marked ballots after being processed by the vote counting device.
Description of measurement (accuracy, cycle time, etc.):
Accuracy – Comparison of ballot and vote totals pre-and post-feed, giving us a yield.
Purpose of data collection:
Ultimately, the goal is to determine if the reliability of the manual vote counting process and ballot counting devices in our municipality will be consistent with our laws requiring a re-count at a 0.5% threshold.
What insight the data will provide:
The data, when counted and compared with the pre-marked ballot totals prior to processing, should tell us if the ballots are distorted in any way when they are fed through the vote counting device such that the outcome (or vote) is altered.
Type of measure (input, process or output):
Process measure.
Type of data (discrete-attribute, discrete-count or continuous):
Discrete-Count.
How it will help the improvement team:
The team will be able to make a decision on whether to eliminate from consideration the possible effects of the ballots being processed through the vote counting device as a possible factor in the overall reliability of the vote counting system.
What will be done with the data after collection:
The team will use the data to arrive at a process accuracy measure, which may be included in the final rolled throughput yield calculation. The team may also use the data to populate a concentration diagram if vote count inaccuracies seem to congregate in one particular area on the ballot that might indicate an obstruction or force in the device that would cause inaccurate vote counts.
Operational Definitions And Methodology
Who? (roles, responsibilities):
Project lead and process owner will supervise/oversee; each team member will participate in the data collection.
What? (define the measure):
Post-feed vote count accuracy: Inaccurate = Post-feed ballot does not match exactly the outcome (votes) of the same pre-marked ballot at pre-feed.
Where? (source, location):
Data collection will take place at the precinct 9 headquarters. Data analysis will be conducted at the State Capital offices.
Scope:
Sampling plan (number of observations):
1,000 total observations are desired. 250 of them coming at each interval.
When (times, intervals, frequencies):
Data collection to take place every Thursday beginning October 9 from 9 a.m. to 10 a.m. Data collection will cease on October 30th.
Past data:
None available.
Present data:
Data collection to begin October 9.
Future data:
To be determined.
How (methodology):
Post-feed vote count accuracy: A pre-marked ballot containing five names written in magic marker (located in the upper right corner of the ballot) will serve as the actual voter intention and will indicate to the participant who they will vote for (i.e. what hole to punch). The participant will take the pre-marked ballot to voting booth A and punch the appropriate holes. The hole-punching will be observed by the team lead or the process wwner. When all the appropriate holes are punched, the team lead or process owner will record the results as they interpret the punches. The participant will then take the ballot and deposit it into the vote counting device. Once the ballot has been fed into the device and the vote has been registered, it will be collected again by the participant and compared to the original, pre-feed vote at booth B. The team lead or process owner will record the results once again as they interpret the punches in their post-feed form. The process will repeat until the desired number of observations has been met.
How (recording data):
Use the tally sheets provided by the team lead. An inaccurate vote count will receive the numeral zero on the tally sheet and an accurate vote count will be recorded (tallied) as the numeral one.
Data Collection (and Measurement) R&R, Accuracy and Stability
Plan for data collection (and measurement) repeatability:
Not applicable.
Plan for data collection (and measurement) reproducibility:
Not applicable.
Plan for measurement systems accuracy:
Not applicable.
Plan for measurement systems stability:
Not applicable.