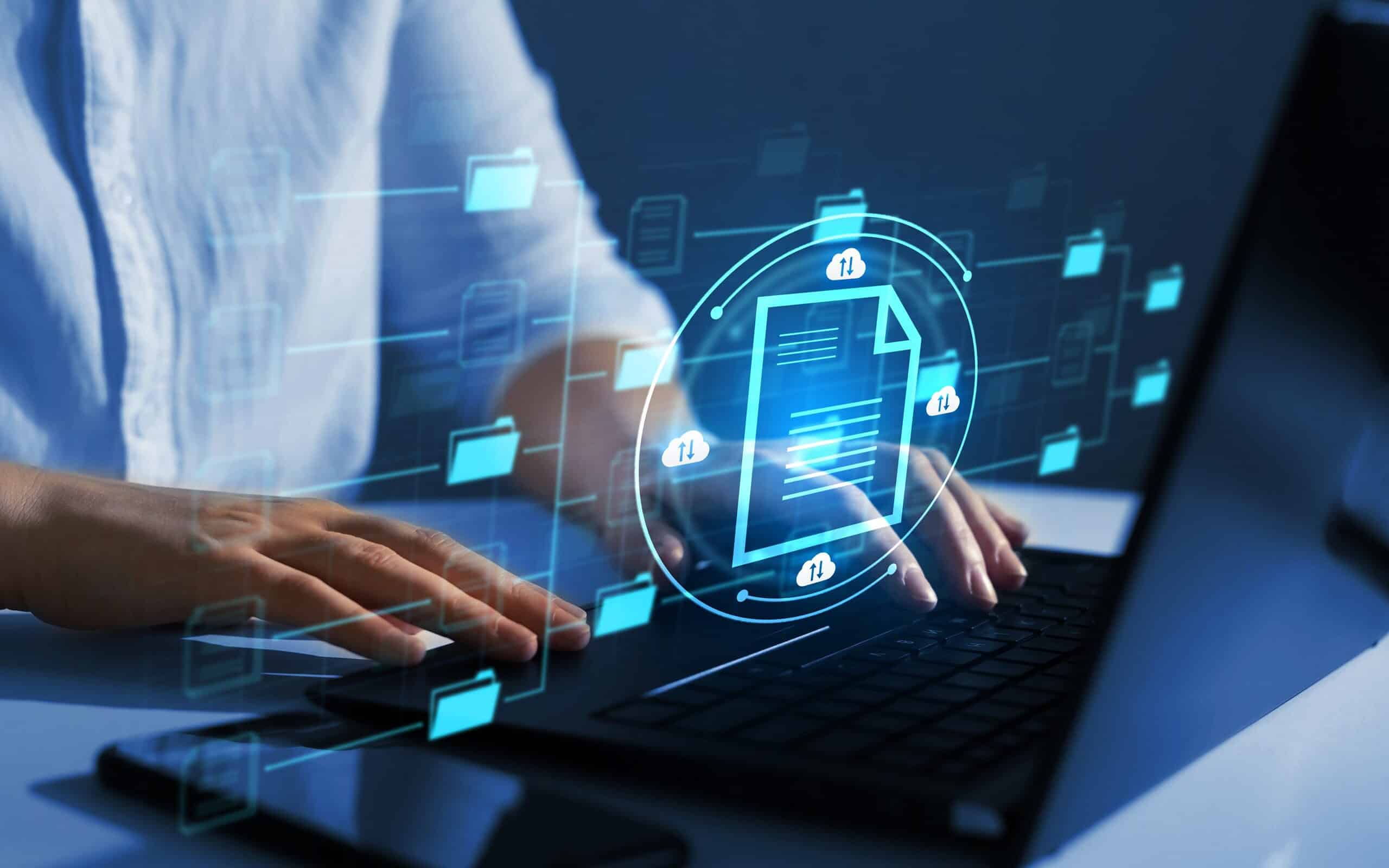
Any third-grade student can tell you whether there is a mathematical difference between two values. But, can they tell you whether that difference is because of random variation or whether it is a statistically significant difference? Probably not.
This article will explain how the p-value will help you decide whether what you are looking at is noise or signal. That is, just random variation or true difference. We will provide you with some of the benefits for knowing and understanding the concept of p-value and some best practices for applying the technique.
Overview: What is the p-value?
Before explaining what the p-value is all about, let’s provide a little context and background to help you better understand what it means and how to interpret it. P-value is used in the context of hypothesis testing, specifically the null hypothesis (Ho).
In a business setting, you’re often faced with a number of questions, such as:
- Is there a difference in the run speed of two machines?
- Is there a difference in invoice processing time between three finance associates?
- Is there a relationship between time spent with the customer and the amount of the sale?
- Is there a relationship between studying for an exam and passing rate?
To determine the answers, you will want to use the statistical technique of hypothesis testing. There are three initial steps you will take when doing a hypothesis test, regardless of the type of question that you are trying to answer.
3 steps to the p-value
First, you must state your Ho. That will always be in the form of the absence of the characteristic in question. For example, if you’re interested in the difference in the average run speed between two machines, you would write the Ho as mu1 – mu2 = 0, where mu represents the population average of the machine speeds for machine 1 and machine 2. In this case, you’re stating that there is an absence of difference (or no difference) between the two machines.
Your second step would be to state your alternate or alternative (Ha) hypothesis. This will be the opposite of your Ho. In this example, your Ha would be written as mu1 – mu2 is not equal to zero.
The third step is your selection of alpha risk. In hypothesis testing, you can make an alpha error. This is rejecting your Ho when you shouldn’t. This is thinking that your new marketing program actually increased sales when in fact, it didn’t. That percent of risk is typically in the range of 1%, 5%, or 10%.
So, where does the p-value fit into all of this? The p-value is your calculated risk of making an alpha error, or claiming success when there really wasn’t any.
To help you determine whether you should go to your boss and declare victory, you will compare your chosen alpha risk with your actual calculated risk and decide what to do next. The rule of thumb is, if the p is low (less than your alpha risk), the Ho should be rejected, but if your p is high (greater than your alpha risk), don’t reject the Ho.
For example, if you chose an alpha risk of 5%, and your calculation of p-value was 1%, then you should march into your boss’s office and proclaim success. Why? Since you are willing to be wrong 5% of the time, but your p-value says you will only be wrong 1% of the time if you reject your Ho, then you will likely go for it and reject your Ho.
But if your p-value was 18%, stay in your own office. Why? Since you were only willing to be wrong 5% of the time when rejecting your Ho, and the p-value says that if you reject your Ho, you will be wrong 18% of the time, common sense says to not reject the null and go back to the drawing board.
3 benefits of understanding the p-value
The p-value is an objective and statistical method for making proper decisions about your process. Relying on the p-value as a guide will help you decide what to do about your Ho.
1. Single value
The p-value is a single calculation that allows you to determine whether any difference you’re seeing is due to random variation or true change.
2. Shows strength of significance
Higher and lower values of the p-value show the relative strength of significance. A higher value shows a greater significance.
3. Easy to use
There are alternative methods for determining whether your difference is significant or not. The two most common are the use of the calculated value of the test statistic and the comparison of it to the critical value of the appropriate distribution and the use of confidence intervals.
The good news is that most statistical software gives you all three, so you can decide what to present given your audience.
Why is the p-value important to consider?
To appreciate the benefits of the p-value, there are a few things you should understand.
Relative guide, not an absolute
The p-value’s usefulness is its simplicity in providing a guide as to whether you should reject or fail to reject your Ho. Don’t get hung up on the actual values but on the values relative to your alpha risk.
Don’t place blind faith in the p-value
While the p-value may indicate whether or not you should reject the Ho, there are probably a number of other factors that may influence your final decision.
What is really significant?
If you reject the Ho using the standard notation of mu1 = mu2 and claim there is a difference, is that difference really meaningful? While there may be a statistical difference, is there really a meaningful difference? If that’s the case, you may decide not to reject the Ho since the difference isn’t large enough to cause you to reject your Ho.
An industry example of the p-value in action
The legal department of a large B2B organization used both internal attorneys and external attorneys to develop, review, and revise contracts with customers. The chief counsel wanted to know whether the internal or external attorneys were more productive and took less time to complete their work.
Data was collected on the time it took for both groups to produce a final contract. The average time it took external attorneys to complete the sample contracts was mathematically faster than the internal group. But was it statistically faster? The Ho was that there was no real difference between the two groups. The Ha was that there was.
The company’s internal statistical resource chose to do a 2-sample t test. The resulting p-value was 0.045, or 4.5%. This was slightly below their chosen 0.05 alpha risk. Going by the book would have guided them to reject the Ho and declare that the outside attorneys were faster. While technically true, the difference was not significant enough to warrant shifting work to the more expensive external legal firm.
3 best practices when thinking about p-value
Your p-value is an outcome of doing a statistical test based on the nature of your data and the question you’re trying to answer. Here are a few things to keep in mind.
1. Underlying assumptions
Statistical tests for hypothesis testing have underlying assumptions. Be sure you’ve met those assumptions, or your p-value — and decision regarding your Ho — may not be valid.
2. Pick your alpha risk before you start
Some people are tempted to select their alpha risk after seeing the results of the p-value calculations. Pick your risk before you start, don’t adjust it after the fact just to get the outcome you want.
3. Select the right test
There are a number of available hypothesis testing tools that. Be sure to properly select the one most appropriate to your question and data.
Frequently Asked Questions (FAQ) about p-value
What does the p-value tell me?
It suggests whether the difference in a sample statistic is due to random noise or to true difference in the population parameter.
How do I use the p-value?
The p-value provides information on whether you should reject your null hypothesis or not.
What values can the p-value be?
The p-value can range between 0 and 1. The higher the value, the more confidence you can have that any difference that you see is real and not just random variation. Of course, your final decision is based on how the p-value compares to your alpha risk.
Summing up the p-value
A p-value is used in hypothesis testing to help you reject or not reject your null hypothesis. The smaller the p-value, the more evidence there is that you should reject your null hypothesis.
P-values are expressed as decimals or percentages and can range from 0 to 1. When you run a hypothesis test, compare your p-value to the alpha risk, which you selected before you ran the test. If your p-value is less than your alpha risk, you have evidence that you can reject your Ho. If your p-value is greater than your alpha risk, it would be best not to reject your Ho.