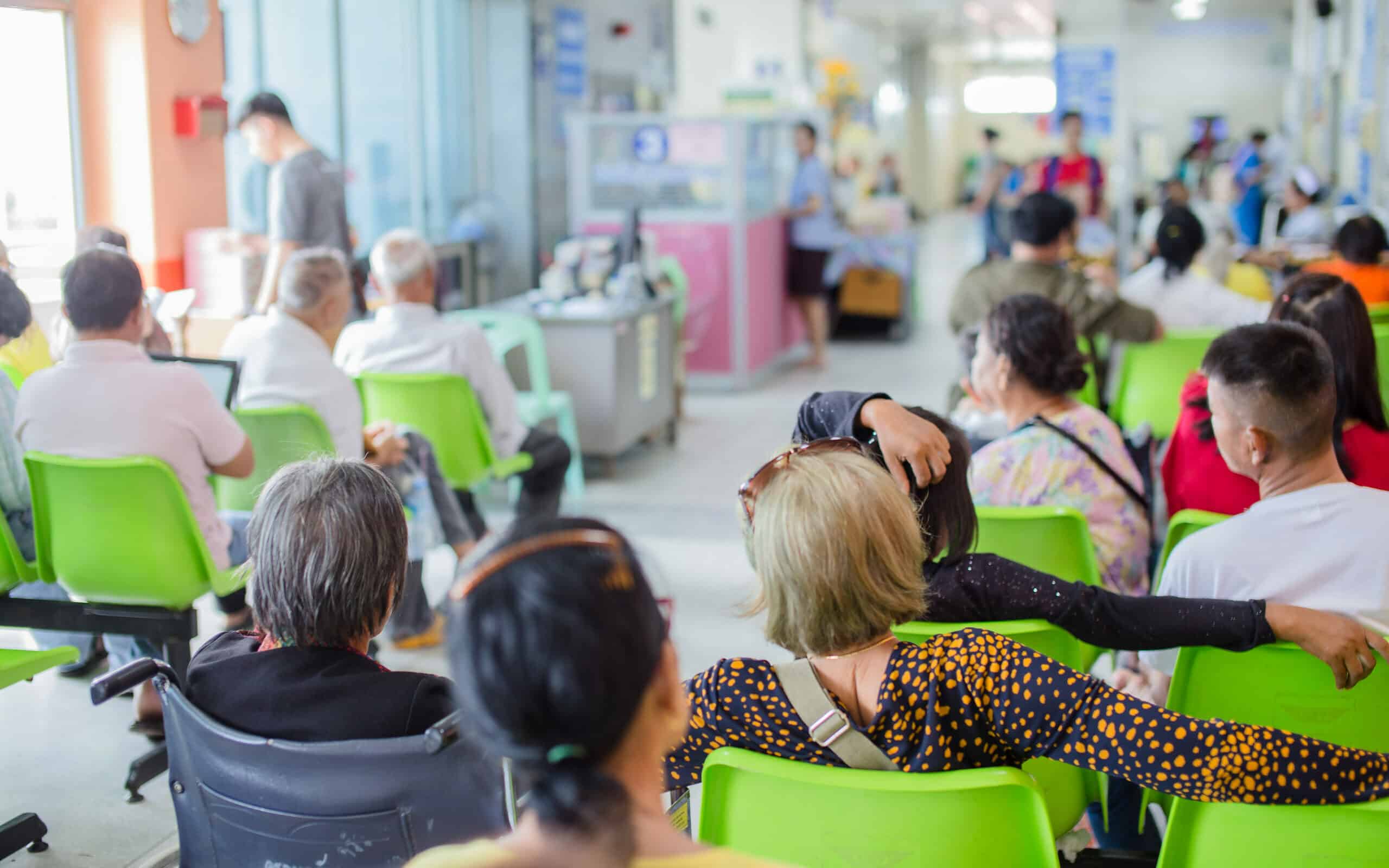
Have you or a family member ever had to visit a hospital Emergency Room? What was your experience? How was your wait time? Let’s see how one hospital handled long wait times and extensive patient dissatisfaction using queuing theory.
The Emergency Room of a public hospital was experiencing long wait times to treat patients.
Wait time in a hospital Emergency Room (ER) is a key performance indicator (KPI) and metric for most hospitals. Besides the moral issue of making patients wait for treatment and pain relief, there is a financial implication as well. This story will be about the issues faced by a hospital we will refer to as the NB Hospital (NBH).
NBH is the flagship hospital for the public hospital system serving the largest county in the state. It is a 500-bed full-service hospital and Level 1 Trauma Center. Given its central location in the county and extensive services, it draws the most emergency patients despite the system having 4 other medical centers. Unfortunately, it has a poor reputation for long wait times when compared to the other medical centers and its main competitor in the adjacent county.
During The Joint Commission assessments, NBH was frequently cited and admonished for poor performance and was directed to reduce their wait times. On average, a visit to the ER could take up to 6 or more hours from entry to triage to treatment and either discharge or admission to the hospital. The Executive Vice President of Operations assigned the task of reducing wait times to Ben, the NBH Director of Performance Improvement, and an experienced Six Sigma Master Black Belt (MBB).
Ben suggested that NBH look at using queuing theory to help manage the line of people waiting for treatment in the ER.
It was already mentioned above that there is a moral obligation to provide treatment and relief to patients as quickly as possible. But there is also the financial impact of long wait times. Although NBH was the first choice of patients, when possible, there were also optional locations in the system and in the competitor’s system in the next county.
If a person entered the ER and it was filled with other waiting patients and no seats to sit in, they often made the decision to leave and seek treatment elsewhere with their doctor or even nearby standalone urgent care facilities. This impacted the revenue and cash flow of NBH. As a public tax supported hospital, they are required to provide free or reduced services to the indigent. NBH has historically operated in the red, so any additional revenue loss was harmful to their financial performance.
First, Ben had to explain the basics of queuing theory so the staff would support his efforts.
Queueing theory has its origins in some 1909 research by Agner Krarup Erlang, who created models to describe the system of incoming calls at the Copenhagen Telephone Exchange Company. These ideas have since seen applications in multiple industries including hospitals.
Once Ben started talking about using queuing theory to try and reduce wait times, it became apparent that most of the staff had no knowledge or experience with the concept and tools. He explained that the basics of queuing theory, as it applied to NBH were:
- The distribution of arrival times of patients to the ER. This is expressed as the term lambda which is defined as the average arrival rate. NBH had little if any impact on when patients decide to come to the ER.
- The service time or, on average, mean time it takes to provide the necessary treatment to the patient. There is great variation in this number given the varied complexity of medical conditions presenting for treatment.
- The number of medical personnel or resources available to provide treatment.
- The queuing discipline. For example; first-in, first-out or last-in, first-out. This would be the prioritization of treating patients. This is often determined in triage where they determine the severity of the patient’s condition and schedule the sequence of treatment.
- The capacity of the queue. You don’t want patients standing outside or backed up in the parking lot. There is also the constraint of available ER treatment rooms and beds.
- The number of patients that need to be treated.
The queuing model Ben wanted to use can be described as a multi-channel, multi-phase model meaning there are several servers (physicians) and a multi-step servicing process (triage to treatment room to ancillary services such as lab tests or radiology and final discharge or admission).
Ben now had to collect the data.
Ben enlisted some of NBH’s Six Sigma Green Belts (GB) to help collect data. Since the ER is a 24/7 operation, there was a lot of data to collect. It was also important to stratify the data by time of day and day of week.since ER volume varied by time and day.
A sampling strategy was devised which included sample size calculations and data collection strategies. The GBs were separated into teams and the data collection process began. The data collection was done over seven days since day-of-week was a factor.
Since lambda is measured in terms of the rate of arrival meaning units per time period, the GBs monitored how many patients arrived at the ER per hour. They also randomly selected patients and tracked how long it took for them from entry to the ER, until they were called to Triage and then how long until they went to a treatment room. Once in the treatment room, the time until a physician showed up was also recorded. Finally, the time was sampled from the treatment room until a patient was fully discharged or was admitted and transported to a room.
Extensive data analysis was conducted to understand the time associated with the steps of the treatment process.
Once all the data was collected, Ben used statistical software to compute the average and standard deviation of the various timed elements from entry to final disposition of the patient. The average overall time from entry to disposition was 6 hours, plus or minus 3 hours depending on the medical condition of the patient and day/time of day.
The calculation of lambda and average service time were done. Using the appropriate queuing formulas, Ben determined that the current arrival rate during most of the time periods exceeded the service rate. This meant that the queue, or number of patients, would theoretically grow to infinity. At some point, patients will abandon the queue and leave to get treatment elsewhere or just go home.
Ben determined that the wait time for triage was reasonable since that is an important determinant of treatment sequencing. The wait time to be moved into a treatment room was longer than benchmark times elsewhere. The average wait time for a physician to appear was 20 minutes. The time to order lab work, x-ray or other services was the most extensive. Once test results were back, the physician was able to prescribe further treatment, discharge, or admission. If a hospital admission was required, there was another long wait for transport, preparation of the room and the necessary paperwork to be completed.
3 best practices to enhance patient satisfaction while they are waiting.
Here are a few hints of what to do if you decide to use queuing theory to reduce the waiting time in your ER and increase patient satisfaction:
Keep the patient busy and informed.
When the patient is distracted while waiting their turn, time seems to pass faster.
You can do this through visual distractions such as displaying content on a TV, digital signage, or information displayed about how much time is left until their turn.
Start the service process as soon as possible.
The self-service / check-in multimedia kiosks serve as an example and give the patient an autonomy that gives them more time and eliminates the need for this to have to go to the service desk and manually fill in information forms. Triage or some initial human interaction should be done as quickly as possible.
Be calm and present accurate information to the best of your ability.
Not all patients will be in a good mood or be extremely polite, especially if they are in a hurry, in pain or have already waited for what they consider a long time. It is necessary to remain calm, sympathetic to their situation, and keep them informed as to what is going on with their case.
These were Ben’s recommendations and alternatives.
Ben and his improvement team explored several options for reducing wait time. Some were quick fixes while others were longer term and would require capital dollars to implement. Here is a list of them:
- Based on arrival rates, be flexible in shifting staff to handle any overload by providing additional service channels. This should reflect the varying volumes by time and day much like McDonald’s does with their staff during lunch, dinner, and other time periods.
- Use a parallel processing rapid medical evaluation approach where, for less critical conditions, treatment begins immediately and includes the initial assessment, the ordering of labs and x-rays, and in some cases, rapid discharge without utilizing a bed in the ER.
- Reduce unnecessary ER visits. Many patients seek instant treatment and won’t wait for an appointment with their primary care physician, or for any required test results to come back. Educate patients so they understand when it’s vital to visit the ER. Increased patient awareness about when they should choose the ER over a doctor’s appointment will reduce unnecessary visits.
- Offering telemedicine is an option. Allowing the ER to provide treatment advice virtually will free up space for more serious needs, reduce unnecessary visits, and therefore decrease waiting times.
- By moving the RNs from triage into direct patient care and making the physician the first point of contact after patient registration, ERs can increase the number of medical professionals providing treatment and, therefore, treat patients quicker. As part of the approach, ERs can utilize lower-cost support staff to care for the kind of less-emergent patient problems normally treated by community doctors in their offices. Doing so further frees up registered nurses to treat the sickest patients.
- Use process mapping of the entire process to identify bottlenecks and non-value-added work. This would eliminate time-consuming and unnecessary work.
- Longer term, additional treatment rooms and an increase in personnel could increase the ER capacity and thus reduce wait times.
- Longer term, a network of smaller urgent care sites could be built to handle the less critical emergencies and reduce the load on NBH.
- Reduce delays for admitted ER patients to be transferred to a hospital bed. Link housekeeping staffing with ER volume so beds and rooms can be made available. This empties beds and treatment rooms for treating other patients.
After implementing several of the above recommendations, the ER waiting time for NBH patients was decreased by 42% with little if any additional costs. The reputation of the hospital and the entire healthcare system improved, as reflected in the Press Ganey surveys given to patients after their visit to NBH.