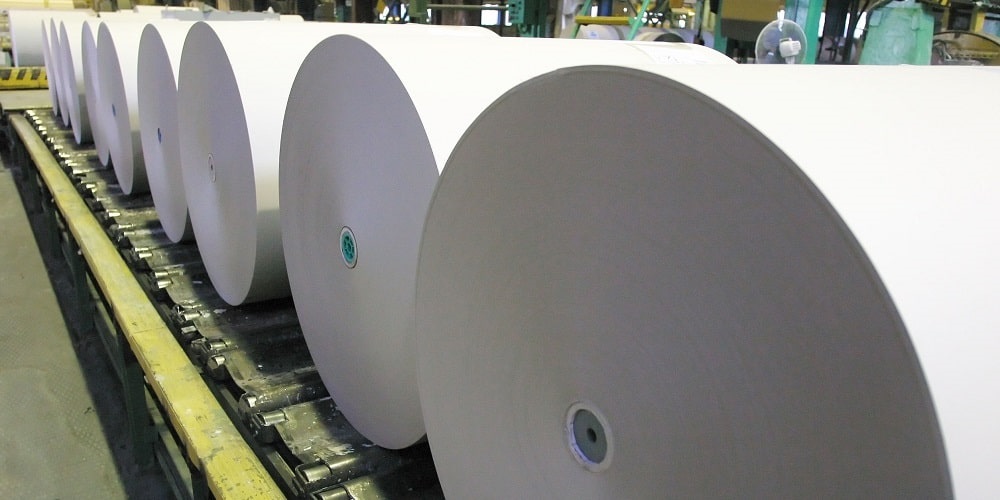
A multinational paper company wanted to reduce its cost of poor quality. The company recognized an opportunity to use Six Sigma concepts to minimize variability in its processes.
Senior management was excited about the idea of applying design of experiments (DOE) to uncover the mathematical relationships among the input and output variables. For them, the possibility of having a y = f(x) equation for their automated equipment meant the potential to run the production line with much improved quality. As is typically the case, however, there were other methods for generating yield improvements, and there were numerous obstacles to a robust application of DOE.
The lack of standardization in the company’s measurement system contributed too much noise to the true equipment response. In addition, the management team was not willing to incur the capacity and yield losses that generally occur during the execution of a DOE. To cap this off, it was later demonstrated that the instability in the process inputs was large enough that it would confound the results of any attempted DOE.
Instead of obtaining the mathematical process model resulting from DOE – what some consider the ultimate Six Sigma solution – the company used a number of other Six Sigma tools to make significant improvements to process yield, with lower costs and in less time. While a typical Six Sigma project of this complexity could last in excess of six months, in this instance the project closed in just six weeks, ending with a plan to leverage the results to other facilities in the company.
Assessing the Measurement System
Although the company had invested a significant amount of capital on the inline process monitoring and control system, it was not realizing expected yield improvements. The production and engineering teams were aware that the control system was being constrained because operators continued to make set point adjustments based on tribal knowledge rather than using the control system to stabilize the process. Initially it was assumed that this was the result of a perception that inline gauges were not properly calibrated or that the gauge responses did not correspond with the results of visual inspection. There was no data, however, to confirm these assumptions, and due to the complexity of the process, creating an intensive, short-term measurement system to gather the necessary data became a cornerstone of the project.
The first step in designing this measurement system was obtaining the voice of the customer. In this case the customer was internal – it was the subsequent production step. This is where product quality was determined, but not where yield had been measured. The existing practice was to measure product quality both inline and manually at the exit of the first piece of production equipment. But ambient temperature, ambient humidity and dwell time were believed to be significant enough to alter the quality of the paper before it reached the next piece of equipment. To effectively relate actual product quality to the inline production equipment data, traceability of the product between production lines was needed. (As a supplemental step in the analysis, correlation models were built among the three output variables.) Additionally, at the onset, scrap data was aggregated over the course of one or more shifts. To enable analysis at granular levels, scrap information needed to be collected on a more frequent basis.
The second step was standardizing the measurement system. Various employees performed visual inspections on each shift at each piece of equipment, using go/no-go gauges. The opportunities to calibrate the gauges to a standard, identify a master inspector and perform a gage R&R analysis were quickly identified. The gage R&R results led to improved inspector training and more accurate inspection data as part of the new measurement system.
Third, there was one input variable (believed to be critical) in the process that was not measured electronically. A standard method for measuring that input variable was instituted along with a specified frequency for manual collection.
Step four in developing the measurement system was ensuring all critical product features were recorded. This would enable rational sub-grouping of any input and output relationships that may be dependent upon product type, time, lot, etc. This data was collected from the production activity records.
The fifth, and final, step was critical – minimizing instrument measurement variation. Where possible, calibrations were performed prior to gathering any data for statistical analysis.
Qualitative Analysis
As this new measurement system was designed and new data collected, other improvement activities were worked on based on qualitative experience and tribal process knowledge. Failure mode and effects analysis and cause-and-effect diagrams were compiled. With these two tools alone, and the subsequent improvement tasks, the team was able to realize a greater than 10 percent reduction in scrap.
Quantitative Data Compilation
Once the system for data collection was defined, the company needed a method to align the various data sources and compile all of the data points into a structured array of y = f(x) observations for analysis. There were three different data sources: 1) the inline process measurements that were stored electronically (from 20 different instruments), 2) two sets of manually recorded visual inspection results (one immediately after the initial production step and one immediately before the subsequent step), and 3) the historical production records, which were also stored electronically.
The observation period necessary for the analysis, calculated based upon historical production volumes of critical product types, was measured in weeks. The variability observed within small time intervals of production dictated relatively small observation periods – in this case, minutes. If observation periods were grouped into larger intervals, the error associated with process sampling or data averaging would confound the process variability.
These factors dictated an extremely large data set, which created a need to automate the process of aligning data points between each of the sets, in time series. For instance, a query of the historical production data generated one record per lot, representing tens of minutes of production, whereas the query of the inline process data generated one record per minute. The company developed an Excel file with worksheet templates for tabulating the data observations, from each of the four sources, and a set of six separate macros to align the observations into a single worksheet (in excess of 20,000 rows of data).
The efficiency of the Excel macros was critical to reduce the lead-time between the observation and analysis phases of the project. This ability to construct the data array in a matter of minutes (versus days) meant the company could periodically review the progress of the observations by performing intermediate analyses. These periodic reviews served to encourage the management team to complete the lengthy observation phase since they were able to continuously review the effectiveness of the approach.
Data Analysis
At the conclusion of the observation period, the final data set was compiled, and Excel and Minitab were used to sort and filter the data into rational subgroups for hypothesis testing. The team was able to apply numerous statistical tools including histograms, run charts, dot plots, t-tests and pareto charts.
At granular levels, relationships were uncovered among the process inputs and the resulting product quality. This enabled the project team to draw data-driven conclusions about the reliability of the inline devices to predict product quality. The representation of data in a visual, statistical format made a tremendous impact in changing the culture of process control from an art of subjectively applying tribal knowledge to an objective, scientific approach with the application of statistical tools.
In the process of performing the statistical analysis, regression attempts demonstrated that special cause variation in the day-to-day operations were too significant, meaning that performing a robust DOE would require a more controlled production environment. So while the approach used in this project did not uncover a mathematical y = f(x) equation for the operation of the production equipment, significant improvements were identified and implemented that resulted in a greater than 20 percent reduction in scrap – a savings of approximately $3-$4 million/year.
Conclusion
A not-uncommon situation: poorly designed data collection methods and perceived constraints in the compilation and analysis of the data prohibit the use of powerful statistical tools such as regression analysis and hypothesis testing. In spite of large capital investment to upgrade controls, install inline measurement devices and automate production lines, the results are less than expected. Even though a facility may have a wealth of process data, it is not being transformed into valuable, actionable information for continuous improvement.
Enabling the application of textbook process improvement tools within these types of environments often requires a unique approach. For this company, unleashing the power of Six Sigma and continuous improvement first required designing the proper system for data collection. The key to success was developing a model of data gathering and compilation, while minimizing disruption to production capacity, but still providing the necessary data set for statistical evaluation. When companies are armed with the right information, making the best choices for improvement are simple.